Open Access
ARTICLE
Noise-Filtering Enhanced Deep Cognitive Diagnosis Model for Latent Skill Discovering
1 National Engineering Research Center of Educational Big Data, Central China Normal University, Wuhan, 430079, China
2 Faculty of Artificial Intelligence in Education, Central China Normal University, Wuhan, 430079, China
3 School of Computer Science and Artificial Intelligence, Wuhan Textile University, Wuhan, 430079, China
* Corresponding Author: Jing Geng. Email:
(This article belongs to the Special Issue: Continual Learning Techniques for Mobile-based Smart Healthcare Information Systems)
Intelligent Automation & Soft Computing 2023, 37(2), 1311-1324. https://doi.org/10.32604/iasc.2023.038481
Received 14 December 2022; Accepted 20 February 2023; Issue published 21 June 2023
A correction of this article was approved in:
Correction: Noise-Filtering Enhanced Deep Cognitive Diagnosis Model for Latent Skill Discovering
Read correction
Abstract
Educational data mining based on student cognitive diagnosis analysis can provide an important decision basis for personalized learning tutoring of students, which has attracted extensive attention from scholars at home and abroad and has made a series of important research progress. To this end, we propose a noise-filtering enhanced deep cognitive diagnosis method to improve the fitting ability of traditional models and obtain students’ skill mastery status by mining the interaction between students and problems nonlinearly through neural networks. First, modeling complex interactions between students and problems with multidimensional features based on cognitive processing theory can enhance the interpretability of the proposed model; second, the neural network is used to predict students’ learning performance, diagnose students’ skill mastery and provide immediate feedback; finally, by comparing the proposed model with several baseline models, extensive experimental results on real data sets demonstrate that the proposed Finally, by comparing the proposed model with several baseline models, the extensive experimental results on the actual data set demonstrate that the proposed model not only improves the accuracy of predicting students’ learning performance but also enhances the interpretability of the neurocognitive diagnostic model.Keywords
Cite This Article
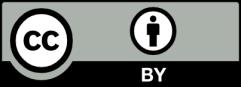
This work is licensed under a Creative Commons Attribution 4.0 International License , which permits unrestricted use, distribution, and reproduction in any medium, provided the original work is properly cited.