Open Access
ARTICLE
A Single Image Derain Method Based on Residue Channel Decomposition in Edge Computing
1 School of Computer Science, Nanjing University of Information Science and Technology, Nanjing, 210044, China
2 School of Automation, Nanjing University of Information Science and Technology, Nanjing, 210044, China
3 School of Geographical Sciences, Nanjing University of Information Science & Technology, Nanjing, 210044, China
4 State Key Laboratory of Resources and Environmental Information System, Institute of Geographic Sciences and Natural Resources Research, Chinese Academy of Sciences, Beijing, 100101, China
5 School of Applied Technology, Nanjing University of Information Science and Technology, Nanjing, 210044, China
* Corresponding Author: Zexuan Yang. Email:
Intelligent Automation & Soft Computing 2023, 37(2), 1469-1482. https://doi.org/10.32604/iasc.2023.038251
Received 04 December 2022; Accepted 10 March 2023; Issue published 21 June 2023
Abstract
The numerous photos captured by low-price Internet of Things (IoT) sensors are frequently affected by meteorological factors, especially rainfall. It causes varying sizes of white streaks on the image, destroying the image texture and ruining the performance of the outdoor computer vision system. Existing methods utilise training with pairs of images, which is difficult to cover all scenes and leads to domain gaps. In addition, the network structures adopt deep learning to map rain images to rain-free images, failing to use prior knowledge effectively. To solve these problems, we introduce a single image derain model in edge computing that combines prior knowledge of rain patterns with the learning capability of the neural network. Specifically, the algorithm first uses Residue Channel Prior to filter out the rainfall textural features then it uses the Feature Fusion Module to fuse the original image with the background feature information. This results in a pre-processed image which is fed into Half Instance Net (HINet) to recover a high-quality rain-free image with a clear and accurate structure, and the model does not rely on any rainfall assumptions. Experimental results on synthetic and real-world datasets show that the average peak signal-to-noise ratio of the model decreases by 0.37 dB on the synthetic dataset and increases by 0.43 dB on the real-world dataset, demonstrating that a combined model reduces the gap between synthetic data and natural rain scenes, improves the generalization ability of the derain network, and alleviates the overfitting problem.Keywords
Cite This Article
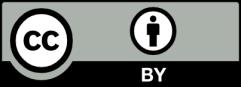