Open Access
ARTICLE
Instance Reweighting Adversarial Training Based on Confused Label
1 Institute of Artificial Intelligence and Blockchain, Guangzhou University, Guangzhou, 511442, China
2 Fujian Provincial Key Laboratory of Information Processing and Intelligent Control, Minjiang University, Fuzhou, 350121, China
* Corresponding Authors: Xianmin Wang. Email: ; Jing Li. Email:
(This article belongs to the Special Issue: AI Powered Human-centric Computing with Cloud/Fog/Edge)
Intelligent Automation & Soft Computing 2023, 37(2), 1243-1256. https://doi.org/10.32604/iasc.2023.038241
Received 03 December 2022; Accepted 24 February 2023; Issue published 21 June 2023
Abstract
Reweighting adversarial examples during training plays an essential role in improving the robustness of neural networks, which lies in the fact that examples closer to the decision boundaries are much more vulnerable to being attacked and should be given larger weights. The probability margin (PM) method is a promising approach to continuously and path-independently measuring such closeness between the example and decision boundary. However, the performance of PM is limited due to the fact that PM fails to effectively distinguish the examples having only one misclassified category and the ones with multiple misclassified categories, where the latter is closer to multi-classification decision boundaries and is supported to be more critical in our observation. To tackle this problem, this paper proposed an improved PM criterion, called confused-label-based PM (CL-PM), to measure the closeness mentioned above and reweight adversarial examples during training. Specifically, a confused label (CL) is defined as the label whose prediction probability is greater than that of the ground truth label given a specific adversarial example. Instead of considering the discrepancy between the probability of the true label and the probability of the most misclassified label as the PM method does, we evaluate the closeness by accumulating the probability differences of all the CLs and ground truth label. CL-PM shares a negative correlation with data vulnerability: data with larger/smaller CL-PM is safer/riskier and should have a smaller/larger weight. Experiments demonstrated that CL-PM is more reliable in indicating the closeness regarding multiple misclassified categories, and reweighting adversarial training based on CL-PM outperformed state-of-the-art counterparts.Keywords
Cite This Article
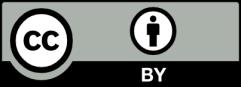