Open Access
ARTICLE
Atrous Convolution-Based Residual Deep CNN for Image Dehazing with Spider Monkey–Particle Swarm Optimization
Department of Electronics and Communication Engineering, VelTech Rangarajan Dr. Sagunthala R&D Institute of Science and Technology, Chennai, 600062, India
* Corresponding Author: CH. Mohan Sai Kumar. Email:
Intelligent Automation & Soft Computing 2023, 37(2), 1711-1728. https://doi.org/10.32604/iasc.2023.038113
Received 28 November 2022; Accepted 13 April 2023; Issue published 21 June 2023
Abstract
Image dehazing is a rapidly progressing research concept to enhance image contrast and resolution in computer vision applications. Owing to severe air dispersion, fog, and haze over the environment, hazy images pose specific challenges during information retrieval. With the advances in the learning theory, most of the learning-based techniques, in particular, deep neural networks are used for single-image dehazing. The existing approaches are extremely computationally complex, and the dehazed images are suffered from color distortion caused by the over-saturation and pseudo-shadow phenomenon. However, the slow convergence rate during training and haze residual is the two demerits in the conventional image dehazing networks. This article proposes a new architecture “Atrous Convolution-based Residual Deep Convolutional Neural Network (CNN)” method with hybrid Spider Monkey-Particle Swarm Optimization for image dehazing. The large receptive field of atrous convolution extracts the global contextual information. The swarm based hybrid optimization is designed for tuning the neural network parameters during training. The experiments over the standard synthetic dataset images used in the proposed network recover clear output images free from distortion and halo effects. It is observed from the statistical analysis that Mean Square Error (MSE) decreases from 74.42 to 62.03 and Peak Signal to Noise Ratio (PSNR) increases from 22.53 to 28.82. The proposed method with hybrid optimization algorithm demonstrates a superior convergence rate and is a more robust than the current state-of-the-art techniques.Keywords
Cite This Article
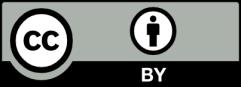
This work is licensed under a Creative Commons Attribution 4.0 International License , which permits unrestricted use, distribution, and reproduction in any medium, provided the original work is properly cited.