Open Access
ARTICLE
Intrusion Detection in the Internet of Things Using Fusion of GRU-LSTM Deep Learning Model
1 Department of Computer Engineering, Prince Sattam Bin Abdulaziz University, Al-Kharj, 16273, Saudi Arabia
2 Department of Computer Engineering, University of Engineering and Technology, Taxila, 47050, Pakistan
3 The FAMLIR Group, The University of Lahore, Lahore, 54000, Pakistan
4 Department of Computer Science, University of Engineering & Technology, Mardan, 23200, Pakistan
5 Faculty of Computing, International Islamic University, Islamabad, 44000, Pakistan
6 EIAS Data Science and Blockchain Lab, College of Computer and Information Sciences, Prince Sultan University, Riyadh, 11586, Saudi Arabia
* Corresponding Author: Zahid Mehmood. Email:
Intelligent Automation & Soft Computing 2023, 37(2), 2279-2290. https://doi.org/10.32604/iasc.2023.037673
Received 12 November 2022; Accepted 04 May 2023; Issue published 21 June 2023
Abstract
Cybersecurity threats are increasing rapidly as hackers use advanced techniques. As a result, cybersecurity has now a significant factor in protecting organizational limits. Intrusion detection systems (IDSs) are used in networks to flag serious issues during network management, including identifying malicious traffic, which is a challenge. It remains an open contest over how to learn features in IDS since current approaches use deep learning methods. Hybrid learning, which combines swarm intelligence and evolution, is gaining attention for further improvement against cyber threats. In this study, we employed a PSO-GA (fusion of particle swarm optimization (PSO) and genetic algorithm (GA)) for feature selection on the CICIDS-2017 dataset. To achieve better accuracy, we proposed a hybrid model called LSTM-GRU of deep learning that fused the GRU (gated recurrent unit) and LSTM (long short-term memory). The results show considerable improvement, detecting several network attacks with 98.86% accuracy. A comparative study with other current methods confirms the efficacy of our proposed IDS scheme.Keywords
Cite This Article
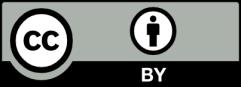
This work is licensed under a Creative Commons Attribution 4.0 International License , which permits unrestricted use, distribution, and reproduction in any medium, provided the original work is properly cited.