Open Access
ARTICLE
Machine Learning Prediction Models of Optimal Time for Aortic Valve Replacement in Asymptomatic Patients
1 Biomedical Engineering Department, The Hashemite University, Zarqa, Jordan
2 Department of Electrical and Computer Engineering, Norwich University, Northfield, Vermont, 05663, USA
3 Department of Biochemistry and Molecular Biology, The Hashemite University, Zarqa, Jordan
4 National Centre for Big Data Science and Artificial Intelligence, Amman, Jordan
* Corresponding Author: Salah Alzghoul. Email:
Intelligent Automation & Soft Computing 2023, 37(1), 455-470. https://doi.org/10.32604/iasc.2023.038338
Received 08 December 2022; Accepted 06 February 2023; Issue published 29 April 2023
Abstract
Currently, the decision of aortic valve replacement surgery time for asymptomatic patients with moderate-to-severe aortic stenosis (AS) is made by healthcare professionals based on the patient’s clinical biometric records. A delay in surgical aortic valve replacement (SAVR) can potentially affect patients’ quality of life. By using ML algorithms, this study aims to predict the optimal SAVR timing and determine the enhancement in moderate-to-severe AS patient survival following surgery. This study represents a novel approach that has the potential to improve decision-making and, ultimately, improve patient outcomes. We analyze data from 176 patients with moderate-to-severe aortic stenosis who had undergone or were indicated for SAVR. We divide the data into two groups: those who died within the first year after SAVR and those who survived for more than one year or were still alive at the last follow-up. We then use six different ML algorithms, Support Vector Machine (SVM), Classification and Regression Tree (C and R tree), Generalized Linear (GL), Chi-Square Automatic Interaction Detector (CHAID), Artificial Neural Network (ANN), and Linear Regression (LR), to generate predictions for the best timing for SAVR. The results showed that the SVM algorithm is the best model for predicting the optimal timing for SAVR and for predicting the post-surgery survival period. By optimizing the timing of SAVR surgery using the SVM algorithm, we observed a significant improvement in the survival period after SAVR. Our study demonstrates that ML algorithms generate reliable models for predicting the optimal timing of SAVR in asymptomatic patients with moderate-to-severe AS.Keywords
Cite This Article
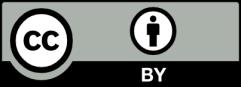
This work is licensed under a Creative Commons Attribution 4.0 International License , which permits unrestricted use, distribution, and reproduction in any medium, provided the original work is properly cited.