Open Access
ARTICLE
Lightweight Method for Plant Disease Identification Using Deep Learning
1 Guangxi Key Lab of Human-machine Interaction and Intelligent Decision, Nanning Normal University, Nanning, 530001, China
2 School of Computer and Information Engineering, Nanning Normal University, Nanning, 530001, China
3 School of Logistics Management and Engineering, Nanning Normal University, Nanning, 530001, China
4 Hangzhou Hikvision Digital Technology Co. Ltd., Hangzhou, 310052, China
* Corresponding Author: Jianbo Lu. Email:
Intelligent Automation & Soft Computing 2023, 37(1), 525-544. https://doi.org/10.32604/iasc.2023.038287
Received 06 December 2022; Accepted 15 February 2023; Issue published 29 April 2023
Abstract
In the deep learning approach for identifying plant diseases, the high complexity of the network model, the large number of parameters, and great computational effort make it challenging to deploy the model on terminal devices with limited computational resources. In this study, a lightweight method for plant diseases identification that is an improved version of the ShuffleNetV2 model is proposed. In the proposed model, the depthwise convolution in the basic module of ShuffleNetV2 is replaced with mixed depthwise convolution to capture crop pest images with different resolutions; the efficient channel attention module is added into the ShuffleNetV2 model network structure to enhance the channel features; and the ReLU activation function is replaced with the ReLU6 activation function to prevent the generation of large gradients. Experiments are conducted on the public dataset PlantVillage. The results show that the proposed model achieves an accuracy of 99.43%, which is an improvement of 0.6 percentage points compared to the ShuffleNetV2 model. Compared to lightweight network models, such as MobileNetV2, MobileNetV3, EfficientNet, and EfficientNetV2, and classical convolutional neural network models, such as ResNet34, ResNet50, and ResNet101, the proposed model has fewer parameters and higher recognition accuracy, which provides guidance for deploying crop pest identification methods on resource-constrained devices, including mobile terminals.Keywords
Cite This Article
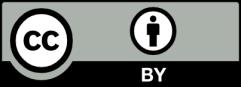