Open Access
ARTICLE
Leaky Cable Fixture Detection in Railway Tunnel Based on RW DCGAN and Compressed GS-YOLOv5
1 School of Information Science and Technology, Shijiazhuang Tiedao University, Shijiazhuang, 050043, China
2 Australian National University, Canberra, 2600, ACT, Australia
* Corresponding Author: Yunzuo Zhang. Email:
Intelligent Automation & Soft Computing 2023, 37(1), 1163-1180. https://doi.org/10.32604/iasc.2023.037902
Received 21 November 2022; Accepted 01 March 2023; Issue published 29 April 2023
Abstract
The communication system of high-speed trains in railway tunnels needs to be built with leaky cables fixed on the tunnel wall with special fixtures. To ensure safety, checking the regular leaky cable fixture is necessary to eliminate the potential danger. At present, the existing fixture detection algorithms are difficult to take into account detection accuracy and speed at the same time. The faulty fixture is also insufficient and difficult to obtain, seriously affecting the model detection effect. To solve these problems, an innovative detection method is proposed in this paper. Firstly, we presented the Res-Net and Wasserstein-Deep Convolution GAN (RW-DCGAN) to implement data augmentation, which can enable the faulty fixture to export more high-quality and irregular images. Secondly, we proposed the Ghost SENet-YOLOv5 (GS-YOLOv5) to enhance the expression of fixture feature, and further improve the detection accuracy and speed. Finally, we adopted the model compression strategy to prune redundant channels, and visualized training details with Grad-CAM to verify the reliability of our model. Experimental results show that the algorithm model is 69.06% smaller than the original YOLOv5 model, with 70.07% fewer parameters, 2.1% higher accuracy and 14.82 fps faster speed, meeting the needs of tunnel fixture detection.Keywords
Cite This Article
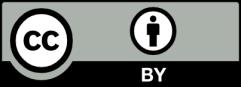