Open Access
ARTICLE
Forecasting the Municipal Solid Waste Using GSO-XGBoost Model
1 B.S. Abdur Rahman Crescent Institute of Science and Technology, GST Road, Vandalur, Chennai, 600048, Tamil Nadu, India
2 Pondicherry University, Chinna Kalapet, Kalapet, Puducherry, 605014, India
* Corresponding Author: Arun Raj Lakshminarayanan. Email:
Intelligent Automation & Soft Computing 2023, 37(1), 301-320. https://doi.org/10.32604/iasc.2023.037823
Received 17 November 2022; Accepted 16 January 2023; Issue published 29 April 2023
Abstract
Waste production rises in tandem with population growth and increased utilization. The indecorous disposal of waste paves the way for huge disaster named as climate change. The National Environment Agency (NEA) of Singapore oversees the sustainable management of waste across the country. The three main contributors to the solid waste of Singapore are paper and cardboard (P&C), plastic, and food scraps. Besides, they have a negligible rate of recycling. In this study, Machine Learning techniques were utilized to forecast the amount of garbage also known as waste audits. The waste audit would aid the authorities to plan their waste infrastructure. The applied models were k-nearest neighbors, Support Vector Regressor, ExtraTrees, CatBoost, and XGBoost. The XGBoost model with its default parameters performed better with a lower Mean Absolute Percentage Error (MAPE) of 8.3093 (P&C waste), 8.3217 (plastic waste), and 6.9495 (food waste). However, Grid Search Optimization (GSO) was used to enhance the parameters of the XGBoost model, increasing its effectiveness. Therefore, the optimized XGBoost algorithm performs the best for P&C, plastics, and food waste with MAPE of 4.9349, 6.7967, and 5.9626, respectively. The proposed GSO-XGBoost model yields better results than the other employed models in predicting municipal solid waste.Keywords
Cite This Article
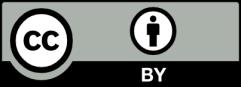
This work is licensed under a Creative Commons Attribution 4.0 International License , which permits unrestricted use, distribution, and reproduction in any medium, provided the original work is properly cited.