Open Access
ARTICLE
Optimizing Spatial Relationships in GCN to Improve the Classification Accuracy of Remote Sensing Images
School of Information Science & Engineering, Shandong Agricultural University, Taian, 271018, China
* Corresponding Author: Feng Zhang. Email:
Intelligent Automation & Soft Computing 2023, 37(1), 491-506. https://doi.org/10.32604/iasc.2023.037558
Received 08 November 2022; Accepted 04 February 2023; Issue published 29 April 2023
Abstract
Semantic segmentation of remote sensing images is one of the core tasks of remote sensing image interpretation. With the continuous development of artificial intelligence technology, the use of deep learning methods for interpreting remote-sensing images has matured. Existing neural networks disregard the spatial relationship between two targets in remote sensing images. Semantic segmentation models that combine convolutional neural networks (CNNs) and graph convolutional neural networks (GCNs) cause a lack of feature boundaries, which leads to the unsatisfactory segmentation of various target feature boundaries. In this paper, we propose a new semantic segmentation model for remote sensing images (called DGCN hereinafter), which combines deep semantic segmentation networks (DSSN) and GCNs. In the GCN module, a loss function for boundary information is employed to optimize the learning of spatial relationship features between the target features and their relationships. A hierarchical fusion method is utilized for feature fusion and classification to optimize the spatial relationship information in the original feature information. Extensive experiments on ISPRS 2D and DeepGlobe semantic segmentation datasets show that compared with the existing semantic segmentation models of remote sensing images, the DGCN significantly optimizes the segmentation effect of feature boundaries, effectively reduces the noise in the segmentation results and improves the segmentation accuracy, which demonstrates the advancements of our model.Keywords
Cite This Article
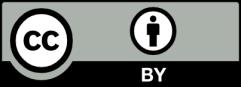