Open Access
ARTICLE
Deep Learning Based Energy Consumption Prediction on Internet of Things Environment
Department of ECE, SRM Institute of Science and Technology, Vadapalani Campus, Vadapalani, 600026, Tamilnadu, India
* Corresponding Author: S. Balaji. Email:
Intelligent Automation & Soft Computing 2023, 37(1), 727-743. https://doi.org/10.32604/iasc.2023.037409
Received 02 November 2022; Accepted 01 February 2023; Issue published 29 April 2023
Abstract
The creation of national energy strategy cannot proceed without accurate projections of future electricity consumption; this is because EC is intimately tied to other forms of energy, such as oil and natural gas. For the purpose of determining and bettering overall energy consumption, there is an urgent requirement for accurate monitoring and calculation of EC at the building level using cutting-edge technology such as data analytics and the internet of things (IoT). Soft computing is a subset of AI that tries to design procedures that are more accurate and reliable, and it has proven to be an effective tool for solving a number of issues that are associated with the use of energy. The use of soft computing for energy prediction is an essential part of the solution to these kinds of challenges. This study presents an improved version of the Harris Hawks Optimization model by combining it with the IHHODL-ECP algorithm for use in Internet of Things settings. The IHHODL-ECP model that has been supplied acts as a useful instrument for the prediction of integrated energy consumption. In order for the raw electrical data to be compatible with the subsequent processing in the IHHODL-ECP model, it is necessary to perform a preprocessing step. The technique of prediction uses a combination of three different kinds of deep learning models, namely DNN, GRU, and DBN. In addition to this, the IHHO algorithm is used as a technique for making adjustments to the hyperparameters. The experimental result analysis of the IHHODL-ECP model is carried out under a variety of different aspects, and the comparison inquiry highlighted the advantages of the IHHODL-ECP model over other present approaches. According to the findings of the experiments conducted with an hourly time resolution, the IHHODL-ECP model obtained a MAPE value of 33.85, which was lower than those produced by the LR, LSTM, and CNN-LSTM models, which had MAPE values of 83.22, 44.57, and 34.62 respectively. These findings provided evidence of the IHHODL-ECP model’s improved ability to provide accurate forecasts.Keywords
Cite This Article
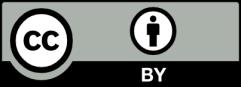
This work is licensed under a Creative Commons Attribution 4.0 International License , which permits unrestricted use, distribution, and reproduction in any medium, provided the original work is properly cited.