Open Access
ARTICLE
FSA-Net: A Cost-efficient Face Swapping Attention Network with Occlusion-Aware Normalization
1 College of Computer Science and Technology, Hengyang Normal University, Hengyang, 421002, China
2 Hunan Provincial Key Laboratory of Intelligent Information Processing and Application, Hengyang, 421002, China
* Corresponding Author: Huihuang Zhao. Email:
Intelligent Automation & Soft Computing 2023, 37(1), 971-983. https://doi.org/10.32604/iasc.2023.037270
Received 28 October 2022; Accepted 04 February 2023; Issue published 29 April 2023
Abstract
The main challenges in face swapping are the preservation and adaptive superimposition of attributes of two images. In this study, the Face Swapping Attention Network (FSA-Net) is proposed to generate photorealistic face swapping. The existing face-swapping methods ignore the blending attributes or mismatch the facial keypoint (cheek, mouth, eye, nose, etc.), which causes artifacts and makes the generated face silhouette non-realistic. To address this problem, a novel reinforced multi-aware attention module, referred to as RMAA, is proposed for handling facial fusion and expression occlusion flaws. The framework includes two stages. In the first stage, a novel attribute encoder is proposed to extract multiple levels of target face attributes and integrate identities and attributes when synthesizing swapped faces. In the second stage, a novel Stochastic Error Refinement (SRE) module is designed to solve the problem of facial occlusion, which is used to repair occlusion regions in a semi-supervised way without any post-processing. The proposed method is then compared with the current state-of-the-art methods. The obtained results demonstrate the qualitative and quantitative outperformance of the proposed method. More details are provided at the footnote link and at .Keywords
Cite This Article
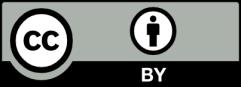
This work is licensed under a Creative Commons Attribution 4.0 International License , which permits unrestricted use, distribution, and reproduction in any medium, provided the original work is properly cited.