Open Access
ARTICLE
Two-Sided Matching Decision Making with Multi-Attribute Probabilistic Hesitant Fuzzy Sets
1 School of Mathematics and Statistics, Heze University, Heze, 274015, China
2 School of Management, Shanghai University of Engineering Science, Shanghai, 201620, China
3 School of Information Management, Jiangxi University of Finance and Economics, Nanchang, 330013, China
* Corresponding Author: Qi Yue. Email:
Intelligent Automation & Soft Computing 2023, 37(1), 849-873. https://doi.org/10.32604/iasc.2023.037090
Received 23 October 2022; Accepted 13 February 2023; Issue published 29 April 2023
Abstract
In previous research on two-sided matching (TSM) decision, agents’ preferences were often given in the form of exact values of ordinal numbers and linguistic phrase term sets. Nowdays, the matching agent cannot perform the exact evaluation in the TSM situations due to the great fuzziness of human thought and the complexity of reality. Probability hesitant fuzzy sets, however, have grown in popularity due to their advantages in communicating complex information. Therefore, this paper develops a TSM decision-making approach with multi-attribute probability hesitant fuzzy sets and unknown attribute weight information. The agent attribute weight vector should be obtained by using the maximum deviation method and Hamming distance. The probabilistic hesitancy fuzzy information matrix of each agent is then arranged to determine the comprehensive evaluation of two matching agent sets. The agent satisfaction degree is calculated using the technique for order preference by similarity to ideal solution (TOPSIS). Additionally, the multi-object programming technique is used to establish a TSM method with the objective of maximizing the agent satisfaction of two-sided agents, and the matching schemes are then established by solving the built model. The study concludes by providing a real-world supply-demand scenario to illustrate the effectiveness of the proposed method. The proposed method is more flexible than prior research since it expresses evaluation information using probability hesitating fuzzy sets and can be used in scenarios when attribute weight information is unclear.Keywords
Cite This Article
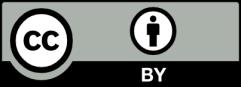