Open Access
ARTICLE
Depth Map Prediction of Occluded Objects Using Structure Tensor with Gain Regularization
Department of Computing Technologies, Faculty of Engineering and Technology, School of Computing, SRM Institute of Science and Technology, Kattankulathur, Chennai, 603203, Tamil Nadu, India
* Corresponding Author: P. Selvaraj. Email:
Intelligent Automation & Soft Computing 2023, 37(1), 1145-1161. https://doi.org/10.32604/iasc.2023.036853
Received 13 October 2022; Accepted 06 December 2022; Issue published 29 April 2023
Abstract
The creation of the 3D rendering model involves the prediction of an accurate depth map for the input images. A proposed approach of a modified semi-global block matching algorithm with variable window size and the gradient assessment of objects predicts the depth map. 3D modeling and view synthesis algorithms could effectively handle the obtained disparity maps. This work uses the consistency check method to find an accurate depth map for identifying occluded pixels. The prediction of the disparity map by semi-global block matching has used the benchmark dataset of Middlebury stereo for evaluation. The improved depth map quality within a reasonable processing time outperforms the other existing depth map prediction algorithms. The experimental results have shown that the proposed depth map predictioncould identify the inter-object boundaryeven with the presence ofocclusion with less detection error and runtime.We observed that the Middlebury stereo dataset has very few images with occluded objects, which made the attainment of gain cumbersome. Considering this gain, we have created our dataset with occlusion using the structured lighting technique. The proposed regularization term as an optimization process in the graph cut algorithm handles occlusion for different smoothing coefficients. The experimented results demonstrated that our dataset had outperformed the Tsukuba dataset regarding the percentage of occluded pixels.Keywords
Cite This Article
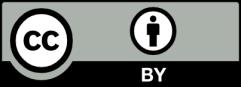
This work is licensed under a Creative Commons Attribution 4.0 International License , which permits unrestricted use, distribution, and reproduction in any medium, provided the original work is properly cited.