Open Access
ARTICLE
P&T-Inf: A Result Inference Method for Context-Sensitive Tasks in Crowdsourcing
1 School of Computer Science and Engineering, Central South University, Changsha, 410075, China
2 Glasgow Caledonian University, Glasgow, G4 0BA, UK
* Corresponding Author: Yan Zhang. Email:
Intelligent Automation & Soft Computing 2023, 37(1), 599-618. https://doi.org/10.32604/iasc.2023.036794
Received 12 October 2022; Accepted 06 January 2023; Issue published 29 April 2023
Abstract
Context-Sensitive Task (CST) is a complex task type in crowdsourcing, such as handwriting recognition, route plan, and audio transcription. The current result inference algorithms can perform well in simple crowdsourcing tasks, but cannot obtain high-quality inference results for CSTs. The conventional method to solve CSTs is to divide a CST into multiple independent simple subtasks for crowdsourcing, but this method ignores the context correlation among subtasks and reduces the quality of result inference. To solve this problem, we propose a result inference algorithm based on the Partially ordered set and Tree augmented naive Bayes Infer (P&T-Inf) for CSTs. Firstly, we screen the candidate results of context-sensitive tasks based on the partially ordered set. If there are parallel candidate sets, the conditional mutual information among subtasks containing context information in external knowledge (such as Google n-gram corpus, American Contemporary English corpus, etc.) will be calculated. Combined with the tree augmented naive (TAN) Bayes model, the maximum weighted spanning tree is used to model the dependencies among subtasks in each CST. We collect two crowdsourcing datasets of handwriting recognition tasks and audio transcription tasks from the real crowdsourcing platform. The experimental results show that our approach improves the quality of result inference in CSTs and reduces the time cost compared with the latest methods.Keywords
Cite This Article
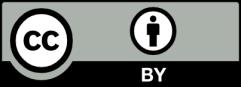
This work is licensed under a Creative Commons Attribution 4.0 International License , which permits unrestricted use, distribution, and reproduction in any medium, provided the original work is properly cited.