Open Access
ARTICLE
Fake News Encoder Classifier (FNEC) for Online Published News Related to COVID-19 Vaccines
1 Department of Computer Science and Information Technology, NED University of Engineering and Technology, Karachi, 75270, Pakistan
2 Department of Computer Science, Iqra University, Karachi, 76400, Pakistan
3 Department of Software Engineering, NED University of Engineering and Technology, Karachi, 75270, Pakistan
* Corresponding Author: Abdul Karim Kazi. Email:
Intelligent Automation & Soft Computing 2023, 37(1), 73-90. https://doi.org/10.32604/iasc.2023.036784
Received 12 October 2022; Accepted 13 December 2022; Issue published 29 April 2023
Abstract
In the past few years, social media and online news platforms have played an essential role in distributing news content rapidly. Consequently. verification of the authenticity of news has become a major challenge. During the COVID-19 outbreak, misinformation and fake news were major sources of confusion and insecurity among the general public. In the first quarter of the year 2020, around 800 people died due to fake news relevant to COVID-19. The major goal of this research was to discover the best learning model for achieving high accuracy and performance. A novel case study of the Fake News Classification using ELECTRA model, which achieved 85.11% accuracy score, is thus reported in this manuscript. In addition to that, a new novel dataset called COVAX-Reality containing COVID-19 vaccine-related news has been contributed. Using the COVAX-Reality dataset, the performance of FNEC is compared to several traditional learning models i.e., Support Vector Machine (SVM), Naive Bayes (NB), Passive Aggressive Classifier (PAC), Long Short-Term Memory (LSTM), Bi-directional LSTM (Bi-LSTM) and Bi-directional Encoder Representations from Transformers (BERT). For the evaluation of FNEC, standard metrics (Precision, Recall, Accuracy, and F1-Score) were utilized.Keywords
Cite This Article
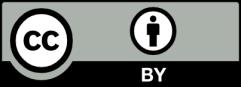
This work is licensed under a Creative Commons Attribution 4.0 International License , which permits unrestricted use, distribution, and reproduction in any medium, provided the original work is properly cited.