Open Access
ARTICLE
MTC: A Multi-Task Model for Encrypted Network Traffic Classification Based on Transformer and 1D-CNN
1 Department of Information Network Security, People’s Public Security University of China, Beijing, 102600, China
2 Safety Precaution Laboratory of Ministry of Public Security, Beijing, 102600, China
* Corresponding Author: Jian Gao. Email:
Intelligent Automation & Soft Computing 2023, 37(1), 619-638. https://doi.org/10.32604/iasc.2023.036701
Received 09 October 2022; Accepted 22 December 2022; Issue published 29 April 2023
Abstract
Traffic characterization (e.g., chat, video) and application identification (e.g., FTP, Facebook) are two of the more crucial jobs in encrypted network traffic classification. These two activities are typically carried out separately by existing systems using separate models, significantly adding to the difficulty of network administration. Convolutional Neural Network (CNN) and Transformer are deep learning-based approaches for network traffic classification. CNN is good at extracting local features while ignoring long-distance information from the network traffic sequence, and Transformer can capture long-distance feature dependencies while ignoring local details. Based on these characteristics, a multi-task learning model that combines Transformer and 1D-CNN for encrypted traffic classification is proposed (MTC). In order to make up for the Transformer’s lack of local detail feature extraction capability and the 1D-CNN’s shortcoming of ignoring long-distance correlation information when processing traffic sequences, the model uses a parallel structure to fuse the features generated by the Transformer block and the 1D-CNN block with each other using a feature fusion block. This structure improved the representation of traffic features by both blocks and allows the model to perform well with both long and short length sequences. The model simultaneously handles multiple tasks, which lowers the cost of training. Experiments reveal that on the ISCX VPN-nonVPN dataset, the model achieves an average F1 score of 98.25% and an average recall of 98.30% for the task of identifying applications, and an average F1 score of 97.94%, and an average recall of 97.54% for the task of traffic characterization. When advanced models on the same dataset are chosen for comparison, the model produces the best results. To prove the generalization, we applied MTC to CICIDS2017 dataset, and our model also achieved good results.Keywords
Cite This Article
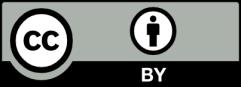