Open Access
ARTICLE
An Improved Granulated Convolutional Neural Network Data Analysis Model for COVID-19 Prediction
1 The Artificial Intelligence College, Chongqing Technology and Business University, Chongqing, 400067, China
2 Chongqing Key Laboratory of IntelliSense and Blockchain Technology, Chongqing, 400067, China
* Corresponding Author: Lianggui Tang. Email:
Intelligent Automation & Soft Computing 2023, 37(1), 179-198. https://doi.org/10.32604/iasc.2023.036684
Received 09 October 2022; Accepted 13 December 2022; Issue published 29 April 2023
Abstract
As COVID-19 poses a major threat to people’s health and economy, there is an urgent need for forecasting methodologies that can anticipate its trajectory efficiently. In non-stationary time series forecasting jobs, there is frequently a hysteresis in the anticipated values relative to the real values. The multilayer deep-time convolutional network and a feature fusion network are combined in this paper’s proposal of an enhanced Multilayer Deep Time Convolutional Neural Network (MDTCNet) for COVID-19 prediction to address this problem. In particular, it is possible to record the deep features and temporal dependencies in uncertain time series, and the features may then be combined using a feature fusion network and a multilayer perceptron. Last but not least, the experimental verification is conducted on the prediction task of COVID-19 real daily confirmed cases in the world and the United States with uncertainty, realizing the short-term and long-term prediction of COVID-19 daily confirmed cases, and verifying the effectiveness and accuracy of the suggested prediction method, as well as reducing the hysteresis of the prediction results.Keywords
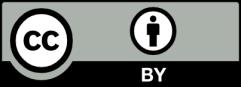