Open Access
ARTICLE
Long-Term Energy Forecasting System Based on LSTM and Deep Extreme Machine Learning
Laboratory of Systems Communications Syscom, National Engineering School of Tunis University of Tunis-El Manar, Tunis, 7000, Tunisia
* Corresponding Author: Cherifa Nakkach. Email:
Intelligent Automation & Soft Computing 2023, 37(1), 545-560. https://doi.org/10.32604/iasc.2023.036385
Received 28 September 2022; Accepted 22 December 2022; Issue published 29 April 2023
Abstract
Due to the development of diversified and flexible building energy resources, the balancing energy supply and demand especially in smart buildings caused an increasing problem. Energy forecasting is necessary to address building energy issues and comfort challenges that drive urbanization and consequent increases in energy consumption. Recently, their management has a great significance as resources become scarcer and their emissions increase. In this article, we propose an intelligent energy forecasting method based on hybrid deep learning, in which the data collected by the smart home through meters is put into the pre-evaluation step. Next, the refined data is the input of a Long Short-Term Memory (LSTM) network, which captures the spatio-temporal correlations from the sequence and generates the feature maps. The output feature map is passed into a Deep Extreme Machine Learning network (with seven hidden layers) for learning, which provides the final prediction. Compared to existing techniques, the LSTM-DELM model offers better prediction results. The simulation values demonstrate the superior performance of the proposed model.Keywords
Cite This Article
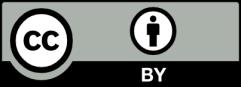
This work is licensed under a Creative Commons Attribution 4.0 International License , which permits unrestricted use, distribution, and reproduction in any medium, provided the original work is properly cited.