Open Access
ARTICLE
An Efficient Approach Based on Remora Optimization Algorithm and Levy Flight for Intrusion Detection
Department of Computer Sciences, Faculty of Computing and Information Technology, Northern Border University, Rafha, 91911, Kingdom of Saudi Arabia
* Corresponding Author: Abdullah Mujawib Alashjaee. Email:
Intelligent Automation & Soft Computing 2023, 37(1), 235-254. https://doi.org/10.32604/iasc.2023.036247
Received 22 September 2022; Accepted 06 January 2023; Issue published 29 April 2023
Abstract
With the recent increase in network attacks by threats, malware, and other sources, machine learning techniques have gained special attention for intrusion detection due to their ability to classify hundreds of features into normal system behavior or an attack attempt. However, feature selection is a vital preprocessing stage in machine learning approaches. This paper presents a novel feature selection-based approach, Remora Optimization Algorithm-Levy Flight (ROA-LF), to improve intrusion detection by boosting the ROA performance with LF. The developed ROA-LF is assessed using several evaluation measures on five publicly available datasets for intrusion detection: Knowledge discovery and data mining tools competition, network security laboratory knowledge discovery and data mining, intrusion detection evaluation dataset, block out traffic network, Canadian institute of cybersecurity and three engineering problems: Cantilever beam design, three-bar truss design, and pressure vessel design. A comparative analysis between developed ROA-LF, particle swarm optimization, salp swarm algorithm, snake optimizer, and the original ROA methods is also presented. The results show that the developed ROA-LF is more efficient and superior to other feature selection methods and the three tested engineering problems for intrusion detection.Keywords
Cite This Article
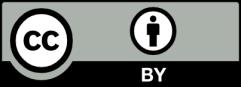
This work is licensed under a Creative Commons Attribution 4.0 International License , which permits unrestricted use, distribution, and reproduction in any medium, provided the original work is properly cited.