Open Access
ARTICLE
Energy Efficient Hyperparameter Tuned Deep Neural Network to Improve Accuracy of Near-Threshold Processor
Department of Electronics and Communication Engineering, Saveetha School of Engineering, Saveetha Institute of Medical and Technical Sciences, Chennai, 602105, Tamilnadu, India
* Corresponding Author: Raghu Gundaala. Email:
Intelligent Automation & Soft Computing 2023, 37(1), 471-489. https://doi.org/10.32604/iasc.2023.036130
Received 18 September 2022; Accepted 13 January 2023; Issue published 29 April 2023
Abstract
When it comes to decreasing margins and increasing energy efficiency in near-threshold and sub-threshold processors, timing error resilience may be viewed as a potentially lucrative alternative to examine. On the other hand, the currently employed approaches have certain restrictions, including high levels of design complexity, severe time constraints on error consolidation and propagation, and uncontaminated architectural registers (ARs). The design of near-threshold circuits, often known as NT circuits, is becoming the approach of choice for the construction of energy-efficient digital circuits. As a result of the exponentially decreased driving current, there was a reduction in performance, which was one of the downsides. Numerous studies have advised the use of NT techniques to chip multiprocessors as a means to preserve outstanding energy efficiency while minimising performance loss. Over the past several years, there has been a clear growth in interest in the development of artificial intelligence hardware with low energy consumption (AI). This has resulted in both large corporations and start-ups producing items that compete on the basis of varying degrees of performance and energy use. This technology’s ultimate goal was to provide levels of efficiency and performance that could not be achieved with graphics processing units or general-purpose CPUs. To achieve this objective, the technology was created to integrate several processing units into a single chip. To accomplish this purpose, the hardware was designed with a number of unique properties. In this study, an Energy Efficient Hyperparameter Tuned Deep Neural Network (EEHPT-DNN) model for Variation-Tolerant Near-Threshold Processor was developed. In order to improve the energy efficiency of artificial intelligence (AI), the EEHPT-DNN model employs several AI techniques. The notion focuses mostly on the repercussions of embedded technologies positioned at the network’s edge. The presented model employs a deep stacked sparse autoencoder (DSSAE) model with the objective of creating a variation-tolerant NT processor. The time-consuming method of modifying hyperparameters through trial and error is substituted with the marine predators optimization algorithm (MPO). This method is utilised to modify the hyperparameters associated with the DSSAE model. To validate that the proposed EEHPT-DNN model has a higher degree of functionality, a full simulation study is conducted, and the results are analysed from a variety of perspectives. This was completed so that the enhanced performance could be evaluated and analysed. According to the results of the study that compared numerous DL models, the EEHPT-DNN model performed significantly better than the other models.Keywords
Cite This Article
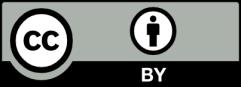
This work is licensed under a Creative Commons Attribution 4.0 International License , which permits unrestricted use, distribution, and reproduction in any medium, provided the original work is properly cited.