Open Access
ARTICLE
CNN-LSTM: A Novel Hybrid Deep Neural Network Model for Brain Tumor Classification
1 Department of Computer Science and Engineering, SRM Institute of Science and Technology, Kattankulathur, 603202, India
2 Department of Computing technologies, SRM Institute of Science and Technology, Kattankulathur, 603202, India
* Corresponding Author: K. M. Umamaheswari. Email:
Intelligent Automation & Soft Computing 2023, 37(1), 1129-1143. https://doi.org/10.32604/iasc.2023.035905
Received 09 September 2022; Accepted 24 February 2023; Issue published 29 April 2023
Abstract
Current revelations in medical imaging have seen a slew of computer-aided diagnostic (CAD) tools for radiologists developed. Brain tumor classification is essential for radiologists to fully support and better interpret magnetic resonance imaging (MRI). In this work, we reported on new observations based on binary brain tumor categorization using HYBRID CNN-LSTM. Initially, the collected image is pre-processed and augmented using the following steps such as rotation, cropping, zooming, CLAHE (Contrast Limited Adaptive Histogram Equalization), and Random Rotation with panoramic stitching (RRPS). Then, a method called particle swarm optimization (PSO) is used to segment tumor regions in an MR image. After that, a hybrid CNN-LSTM classifier is applied to classify an image as a tumor or normal. In this proposed hybrid model, the CNN classifier is used for generating the feature map and the LSTM classifier is used for the classification process. The effectiveness of the proposed approach is analyzed based on the different metrics and outcomes compared to different methods.Keywords
Cite This Article
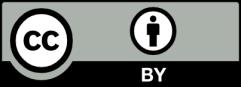