Open Access
ARTICLE
Power Information System Database Cache Model Based on Deep Machine Learning
Kyonggi University, Suwon, 449-701, Korea
* Corresponding Author: Manjiang Xing. Email:
Intelligent Automation & Soft Computing 2023, 37(1), 1081-1090. https://doi.org/10.32604/iasc.2023.034750
Received 26 July 2022; Accepted 13 December 2022; Issue published 29 April 2023
Abstract
At present, the database cache model of power information system has problems such as slow running speed and low database hit rate. To this end, this paper proposes a database cache model for power information systems based on deep machine learning. The caching model includes program caching, Structured Query Language (SQL) preprocessing, and core caching modules. Among them, the method to improve the efficiency of the statement is to adjust operations such as multi-table joins and replacement keywords in the SQL optimizer. Build predictive models using boosted regression trees in the core caching module. Generate a series of regression tree models using machine learning algorithms. Analyze the resource occupancy rate in the power information system to dynamically adjust the voting selection of the regression tree. At the same time, the voting threshold of the prediction model is dynamically adjusted. By analogy, the cache model is re-initialized. The experimental results show that the model has a good cache hit rate and cache efficiency, and can improve the data cache performance of the power information system. It has a high hit rate and short delay time, and always maintains a good hit rate even under different computer memory; at the same time, it only occupies less space and less CPU during actual operation, which is beneficial to power The information system operates efficiently and quickly.Keywords
Cite This Article
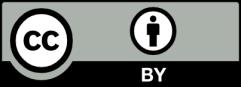
This work is licensed under a Creative Commons Attribution 4.0 International License , which permits unrestricted use, distribution, and reproduction in any medium, provided the original work is properly cited.