Open Access
ARTICLE
Semi-Supervised Clustering Algorithm Based on Deep Feature Mapping
1 Southwest China Institute of Electronic Technology, Chengdu, 610036, China
2 School of Mathematics, Southwest Jiaotong University, Chengdu, 611756, China
* Corresponding Author: Chun Zhou. Email:
Intelligent Automation & Soft Computing 2023, 37(1), 815-831. https://doi.org/10.32604/iasc.2023.034656
Received 23 July 2022; Accepted 13 December 2022; Issue published 29 April 2023
Abstract
Clustering analysis is one of the main concerns in data mining. A common approach to the clustering process is to bring together points that are close to each other and separate points that are away from each other. Therefore, measuring the distance between sample points is crucial to the effectiveness of clustering. Filtering features by label information and measuring the distance between samples by these features is a common supervised learning method to reconstruct distance metric. However, in many application scenarios, it is very expensive to obtain a large number of labeled samples. In this paper, to solve the clustering problem in the few supervised sample and high data dimensionality scenarios, a novel semi-supervised clustering algorithm is proposed by designing an improved prototype network that attempts to reconstruct the distance metric in the sample space with a small amount of pairwise supervised information, such as Must-Link and Cannot-Link, and then cluster the data in the new metric space. The core idea is to make the similar ones closer and the dissimilar ones further away through embedding mapping. Extensive experiments on both real-world and synthetic datasets show the effectiveness of this algorithm. Average clustering metrics on various datasets improved by 8% compared to the comparison algorithm.Keywords
Cite This Article
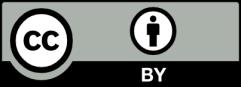
This work is licensed under a Creative Commons Attribution 4.0 International License , which permits unrestricted use, distribution, and reproduction in any medium, provided the original work is properly cited.