Open Access
ARTICLE
Intrusion Detection System Through Deep Learning in Routing MANET Networks
1
Department Electrical and Computer Engineering, Altinbas University, Istanbul, Turkey
2
Department Computer Technology Engineering, Al-Esraa University, Baghdad, Iraq
3
Electric, Autonomous and Unmanned Vehicles Application and Research Centre, Altinbas University, Istanbul, Turkey
4
Faculty of Engineering and Architecture, Altinbas University, Istanbul, Turkey
5
Department Electrical and Electronics Engineering, Ege University, Izmir, Turkey
* Corresponding Author: Zainab Ali Abbood. Email:
Intelligent Automation & Soft Computing 2023, 37(1), 269-281. https://doi.org/10.32604/iasc.2023.035276
Received 15 August 2022; Accepted 04 November 2022; Issue published 29 April 2023
Abstract
Deep learning (DL) is a subdivision of machine learning (ML) that employs numerous algorithms, each of which provides various explanations of the data it consumes; mobile ad-hoc networks (MANET) are growing in prominence. For reasons including node mobility, due to MANET’s potential to provide small-cost solutions for real-world contact challenges, decentralized management, and restricted bandwidth, MANETs are more vulnerable to security threats. When protecting MANETs from attack, encryption and authentication schemes have their limits. However, deep learning (DL) approaches in intrusion detection systems (IDS) can adapt to the changing environment of MANETs and allow a system to make intrusion decisions while learning about its mobility in the environment. IDSs are a secondary defiance system for mobile ad-hoc networks vs. attacks since they monitor network traffic and report anything unusual. Recently, many scientists have employed deep neural networks (DNNs) to address intrusion detection concerns. This paper used MANET to recognize complex patterns by focusing on security standards through efficiency determination and identifying malicious nodes, and mitigating network attacks using the three algorithms presented Cascading Back Propagation Neural Network (CBPNN), Feedforward-Neural-Network (FNN), and Cascading-Back-Propagation-NeuralNetwork (CBPNN) (FFNN). In addition to Convolutional-Neural-Network (CNN), these primary forms of deep neural network (DNN) building designs are widely used to improve the performance of intrusion detection systems (IDS) and the use of IDS in conjunction with machine learning (ML). Furthermore, machine learning (ML) techniques than their statistical and logical methods provide MANET network learning capabilities and encourage adaptation to different environments. Compared with another current model, The proposed model has better average receiving packet (ARP) and end-to-end (E2E) performance. The results have been obtained from CBP, FFNN and CNN 74%, 82% and 85%, respectively, by the time (27, 18, and 17 s).Keywords
Cite This Article
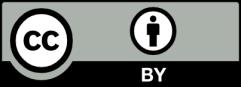
This work is licensed under a Creative Commons Attribution 4.0 International License , which permits unrestricted use, distribution, and reproduction in any medium, provided the original work is properly cited.