Open Access
ARTICLE
Performance Analysis of Intrusion Detection System in the IoT Environment Using Feature Selection Technique
Department of Computer Science, College of Computer, Qassim University, Saudi Arabia
* Corresponding Author: Khalil Hamdi Ateyeh Al-Shqeerat. Email:
Intelligent Automation & Soft Computing 2023, 36(3), 3709-3724. https://doi.org/10.32604/iasc.2023.036856
Received 13 October 2022; Accepted 13 December 2022; Issue published 15 March 2023
Abstract
The increasing number of security holes in the Internet of Things (IoT) networks creates a question about the reliability of existing network intrusion detection systems. This problem has led to the developing of a research area focused on improving network-based intrusion detection system (NIDS) technologies. According to the analysis of different businesses, most researchers focus on improving the classification results of NIDS datasets by combining machine learning and feature reduction techniques. However, these techniques are not suitable for every type of network. In light of this, whether the optimal algorithm and feature reduction techniques can be generalized across various datasets for IoT networks remains. The paper aims to analyze the methods used in this research and whether they can be generalized to other datasets. Six ML models were used in this study, namely, logistic regression (LR), decision trees (DT), Naive Bayes (NB), random forest (RF), K-nearest neighbors (KNN), and linear SVM. The primary detection algorithms used in this study, Principal Component (PCA) and Gini Impurity-Based Weighted Forest (GIWRF) evaluated against three global ToN-IoT datasets, UNSW-NB15, and Bot-IoT datasets. The optimal number of dimensions for each dataset was not studied by applying the PCA algorithm. It is stated in the paper that the selection of datasets affects the performance of the FE techniques and detection algorithms used. Increasing the efficiency of this research area requires a comprehensive standard feature set that can be used to improve quality over time.Keywords
Cite This Article
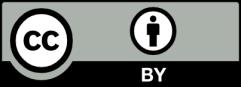
This work is licensed under a Creative Commons Attribution 4.0 International License , which permits unrestricted use, distribution, and reproduction in any medium, provided the original work is properly cited.