Open Access
ARTICLE
Data Masking for Chinese Electronic Medical Records with Named Entity Recognition
1 School of Computer Science, Nanjing University of Information Science and Technology, Nanjing, 21000, China
2 Geospatial Information Engineering Research Center, Xinjiang Production and Construction Corps, Shihezi, 832003, China
3 College of Big Data and Intelligent Engineering, Southwest Forestry University, Kunming, 650224, China
* Corresponding Author: Xiaolong Xu. Email:
Intelligent Automation & Soft Computing 2023, 36(3), 3657-3673. https://doi.org/10.32604/iasc.2023.036831
Received 13 October 2022; Accepted 13 December 2022; Issue published 15 March 2023
Abstract
With the rapid development of information technology, the electronification of medical records has gradually become a trend. In China, the population base is huge and the supporting medical institutions are numerous, so this reality drives the conversion of paper medical records to electronic medical records. Electronic medical records are the basis for establishing a smart hospital and an important guarantee for achieving medical intelligence, and the massive amount of electronic medical record data is also an important data set for conducting research in the medical field. However, electronic medical records contain a large amount of private patient information, which must be desensitized before they are used as open resources. Therefore, to solve the above problems, data masking for Chinese electronic medical records with named entity recognition is proposed in this paper. Firstly, the text is vectorized to satisfy the required format of the model input. Secondly, since the input sentences may have a long or short length and the relationship between sentences in context is not negligible. To this end, a neural network model for named entity recognition based on bidirectional long short-term memory (BiLSTM) with conditional random fields (CRF) is constructed. Finally, the data masking operation is performed based on the named entity recognition results, mainly using regular expression filtering encryption and principal component analysis (PCA) word vector compression and replacement. In addition, comparison experiments with the hidden markov model (HMM) model, LSTM-CRF model, and BiLSTM model are conducted in this paper. The experimental results show that the method used in this paper achieves 92.72% Accuracy, 92.30% Recall, and 92.51% F1_score, which has higher accuracy compared with other models.Keywords
Cite This Article
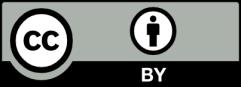
This work is licensed under a Creative Commons Attribution 4.0 International License , which permits unrestricted use, distribution, and reproduction in any medium, provided the original work is properly cited.