Open Access
ARTICLE
Multi-Task Deep Learning with Task Attention for Post-Click Conversion Rate Prediction
School of Information Science and Engineering, Yunnan University, Kunming, 650500, China
* Corresponding Author: Xiaobing Zhou. Email:
Intelligent Automation & Soft Computing 2023, 36(3), 3583-3593. https://doi.org/10.32604/iasc.2023.036622
Received 07 October 2022; Accepted 06 December 2022; Issue published 15 March 2023
Abstract
Online advertising has gained much attention on various platforms as a hugely lucrative market. In promoting content and advertisements in real life, the acquisition of user target actions is usually a multi-step process, such as impression→click→conversion, which means the process from the delivery of the recommended item to the user’s click to the final conversion. Due to data sparsity or sample selection bias, it is difficult for the trained model to achieve the business goal of the target campaign. Multi-task learning, a classical solution to this problem, aims to generalize better on the original task given several related tasks by exploiting the knowledge between tasks to share the same feature and label space. Adaptively learned task relations bring better performance to make full use of the correlation between tasks. We train a general model capable of capturing the relationships between various tasks on all existing active tasks from a meta-learning perspective. In addition, this paper proposes a Multi-task Attention Network (MAN) to identify commonalities and differences between tasks in the feature space. The model performance is improved by explicitly learning the stacking of task relationships in the label space. To illustrate the effectiveness of our method, experiments are conducted on Alibaba Click and Conversion Prediction (Ali-CCP) dataset. Experimental results show that the method outperforms the state-of-the-art multi-task learning methods.Keywords
Cite This Article
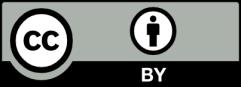
This work is licensed under a Creative Commons Attribution 4.0 International License , which permits unrestricted use, distribution, and reproduction in any medium, provided the original work is properly cited.