Open Access
ARTICLE
The IOMT-Based Risk-Free Approach to Lung Disorders Detection from Exhaled Breath Examination
Department of Artificial Intelligence, Faculty of Computing, The Islamia University of Bahawalpur, Bahawalpur, 63100, Pakistan
* Corresponding Author: Ghulam Gilanie. Email:
Intelligent Automation & Soft Computing 2023, 36(3), 2835-2847. https://doi.org/10.32604/iasc.2023.034857
Received 29 July 2022; Accepted 14 November 2022; Issue published 15 March 2023
Abstract
The lungs are the main fundamental part of the human respiratory system and are among the major organs of the human body. Lung disorders, including Coronavirus (Covid-19), are among the world’s deadliest and most life-threatening diseases. Early and social distance-based detection and treatment can save lives as well as protect the rest of humanity. Even though X-rays or Computed Tomography (CT) scans are the imaging techniques to analyze lung-related disorders, medical practitioners still find it challenging to analyze and identify lung cancer from scanned images. unless COVID-19 reaches the lungs, it is unable to be diagnosed. through these modalities. So, the Internet of Medical Things (IoMT) and machine learning-based computer-assisted approaches have been developed and applied to automate these diagnostic procedures. This study also aims at investigating an automated approach for the detection of COVID-19 and lung disorders other than COVID-19 infection in a non-invasive manner at their early stages through the analysis of human breath. Human breath contains several volatile organic compounds, i.e., water vapor (5.0%–6.3%), nitrogen (79%), oxygen (13.6%–16.0%), carbon dioxide (4.0%–5.3%), argon (1%), hydrogen (1 ppm) (parts per million), carbon monoxide (1%), proteins (1%), isoprene (1%), acetone (1%), and ammonia (1%). Beyond these limits, the presence of a certain volatile organic compound (VOC) may indicate a disease. The proposed research not only aims to increase the accuracy of lung disorder detection from breath analysis but also to deploy the model in a real-time environment as a home appliance. Different sensors detect VOC; microcontrollers and machine learning models have been used to detect these lung disorders. Overall, the suggested methodology is accurate, efficient, and non-invasive. The proposed method obtained an accuracy of 93.59%, a sensitivity of 89.59%, a specificity of 94.87%, and an AUC-Value of 0.96.Keywords
Cite This Article
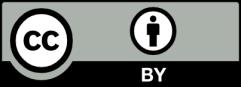
This work is licensed under a Creative Commons Attribution 4.0 International License , which permits unrestricted use, distribution, and reproduction in any medium, provided the original work is properly cited.