Open Access
ARTICLE
Applied Linguistics with Mixed Leader Optimizer Based English Text Summarization Model
1 Department of Applied Linguistics, College of Languages, Princess Nourah Bint Abdulrahman University, P.O. Box 84428, Riyadh, 11671, Saudi Arabia
2 Department of Computer Sciences, College of Computing and Information System, Umm Al-Qura University, Saudi Arabia
3 Department of Information Systems, Faculty of Computing and Information Technology, King Abdulaziz University, Jeddah, Saudi Arabia
4 Research Centre, Future University in Egypt, New Cairo, 11845, Egypt
5 Department of Computer and Self Development, Preparatory Year Deanship, Prince Sattam bin Abdulaziz University, AlKharj, Saudi Arabia
6 Department of English, College of Science & Humanities, Prince Sattam bin Abdulaziz University, AlKharj, Saudi Arabia
* Corresponding Author: Manar Ahmed Hamza. Email:
Intelligent Automation & Soft Computing 2023, 36(3), 3203-3219. https://doi.org/10.32604/iasc.2023.034848
Received 29 July 2022; Accepted 23 November 2022; Issue published 15 March 2023
Abstract
The term ‘executed linguistics’ corresponds to an interdisciplinary domain in which the solutions are identified and provided for real-time language-related problems. The exponential generation of text data on the Internet must be leveraged to gain knowledgeable insights. The extraction of meaningful insights from text data is crucial since it can provide value-added solutions for business organizations and end-users. The Automatic Text Summarization (ATS) process reduces the primary size of the text without losing any basic components of the data. The current study introduces an Applied Linguistics-based English Text Summarization using a Mixed Leader-Based Optimizer with Deep Learning (ALTS-MLODL) model. The presented ALTS-MLODL technique aims to summarize the text documents in the English language. To accomplish this objective, the proposed ALTS-MLODL technique pre-processes the input documents and primarily extracts a set of features. Next, the MLO algorithm is used for the effectual selection of the extracted features. For the text summarization process, the Cascaded Recurrent Neural Network (CRNN) model is exploited whereas the Whale Optimization Algorithm (WOA) is used as a hyperparameter optimizer. The exploitation of the MLO-based feature selection and the WOA-based hyperparameter tuning enhanced the summarization results. To validate the performance of the ALTS-MLODL technique, numerous simulation analyses were conducted. The experimental results signify the superiority of the proposed ALTS-MLODL technique over other approaches.Keywords
Cite This Article
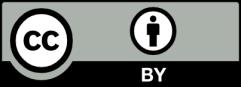