Open Access
ARTICLE
Improved Fruitfly Optimization with Stacked Residual Deep Learning Based Email Classification
1 Department of Applied Linguistics, College of Languages, Princess Nourah bint Abdulrahman University, P.O. Box 84428, Riyadh, 11671, Saudi Arabia
2 Department of Computer Sciences, College of Computing and Information System, Umm Al-Qura University, Makkah, 24211, Saudi Arabia
3 Department of Information Systems, Faculty of Computing and Information Technology, King Abdulaziz University, Jeddah, 22230, Saudi Arabia
4 Research Centre, Future University in Egypt, New Cairo, 11845, Egypt
5 Department of Computer and Self Development, Preparatory Year Deanship, Prince Sattam bin Abdulaziz University, AlKharj, 16242, Saudi Arabia
6 Department of English, College of Science & Humanities, Prince Sattam bin Abdulaziz University, AlKharj, 16242, Saudi Arabia
* Corresponding Author: Abdelwahed Motwakel. Email:
Intelligent Automation & Soft Computing 2023, 36(3), 3139-3155. https://doi.org/10.32604/iasc.2023.034841
Received 29 July 2022; Accepted 26 October 2022; Issue published 15 March 2023
Abstract
Applied linguistics means a wide range of actions which include addressing a few language-based problems or solving some language-based concerns. Emails stay in the leading positions for business as well as personal use. This popularity grabs the interest of individuals with malevolent intentions—phishing and spam email assaults. Email filtering mechanisms were developed incessantly to follow unwanted, malicious content advancement to protect the end-users. But prevailing solutions were focused on phishing email filtering and spam and whereas email labelling and analysis were not fully advanced. Thus, this study provides a solution related to email message body text automatic classification into phishing and email spam. This paper presents an Improved Fruitfly Optimization with Stacked Residual Recurrent Neural Network (IFFO-SRRNN) based on Applied Linguistics for Email Classification. The presented IFFO-SRRNN technique examines the intrinsic features of email for the identification of spam emails. At the preliminary level, the IFFO-SRRNN model follows the email pre-processing stage to make it compatible with further computation. Next, the SRRNN method can be useful in recognizing and classifying spam emails. As hyperparameters of the SRRNN model need to be effectually tuned, the IFFO algorithm can be utilized as a hyperparameter optimizer. To investigate the effectual email classification results of the IFFO-SRDL technique, a series of simulations were taken placed on public datasets, and the comparison outcomes highlight the enhancements of the IFFO-SRDL method over other recent approaches with an accuracy of 98.86%.Keywords
Cite This Article
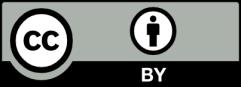