Open Access
ARTICLE
Gender Identification Using Marginalised Stacked Denoising Autoencoders on Twitter Data
1 Department of Language Preparation, Arabic Language Teaching Institute, Princess Nourah Bint Abdulrahman University, P.O. Box 84428, Riyadh, 11671, Saudi Arabia
2 Department of Computer Sciences, College of Computing and Information System, Umm Al-Qura University, Makkah, 24211, Saudi Arabia
3 Department of Teachers Training, Arabic Linguistics Institute, King Saud University, P.O. BOX 145111, Riyadh, ZIP 4545, Saudi Arabia
4 Department of Computer Science, College of Sciences and Humanities-Aflaj, Prince Sattam bin Abdulaziz University, Al-Aflaj, 16733, Saudi Arabia
5 Department of Computer Science, Faculty of Computers and Information Technology, Future University in Egypt, New Cairo, 11835, Egypt
6 Department of Computer and Self Development, Preparatory Year Deanship, Prince Sattam bin Abdulaziz University, AlKharj, Saudi Arabia
* Corresponding Author: Mesfer Al Duhayyim. Email:
Intelligent Automation & Soft Computing 2023, 36(3), 2529-2544. https://doi.org/10.32604/iasc.2023.034623
Received 22 July 2022; Accepted 22 September 2022; Issue published 15 March 2023
Abstract
Gender analysis of Twitter could reveal significant socio-cultural differences between female and male users. Efforts had been made to analyze and automatically infer gender formerly for more commonly spoken languages’ content, but, as we now know that limited work is being undertaken for Arabic. Most of the research works are done mainly for English and least amount of effort for non-English language. The study for Arabic demographic inference like gender is relatively uncommon for social networking users, especially for Twitter. Therefore, this study aims to design an optimal marginalized stacked denoising autoencoder for gender identification on Arabic Twitter (OMSDAE-GIAT) model. The presented OMSDAE-GIAR technique mainly concentrates on the identification and classification of gender exist in the Twitter data. To attain this, the OMSDAE-GIAT model derives initial stages of data pre-processing and word embedding. Next, the MSDAE model is exploited for the identification of gender into two classes namely male and female. In the final stage, the OMSDAE-GIAT technique uses enhanced bat optimization algorithm (EBOA) for parameter tuning process, showing the novelty of our work. The performance validation of the OMSDAE-GIAT model is inspected against an Arabic corpus dataset and the results are measured under distinct metrics. The comparison study reported the enhanced performance of the OMSDAE-GIAT model over other recent approaches.Keywords
Cite This Article
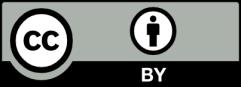