Open Access
ARTICLE
Data-Driven Probabilistic System for Batsman Performance Prediction in a Cricket Match
1 Superior University, Lahore, 54000, Pakistan
2 Intelligent Data Visual Computing Research (IDVCR), Lahore, Pakistan
3 National University of Technology, Islamabad, 44000, Pakistan
* Corresponding Author: Fawad Nasim. Email:
Intelligent Automation & Soft Computing 2023, 36(3), 2865-2877. https://doi.org/10.32604/iasc.2023.034258
Received 12 July 2022; Accepted 23 November 2022; Issue published 15 March 2023
Abstract
Batsmen are the backbone of any cricket team and their selection is very critical to the team’s success. A good batsman not only scores run but also provides stability to the team’s innings. The most important factor in selecting a batsman is their ability to score runs. It is a generally accepted notion that the future performance of a batsman can be predicted by observing and analyzing their past record. This hypothesis is based on the fact that a player’s batting average is generally considered to be a good indicator of their future performance. We proposed a data-driven probabilistic system for batsman performance prediction in the game of cricket. It captures the dependencies between the runs scored by a batsman in consecutive balls. The system is evaluated using a dataset extracted from the Cricinfo website. The system is based on a Hidden Markov model (HMM). HMM is used to generate the prediction model to foresee players’ upcoming performances. The first-order Markov chain assumes that the probability of a batsman scoring runs in the next ball is only dependent on how many runs he scored in the current ball. We use a data-driven approach to learn the parameters of the HMM from data. A probabilistic matrix is made that predicts what scores the batter can do on the upcoming balls. The results show that the system can accurately predict the runs scored by a batsman in a ball.Keywords
Cite This Article
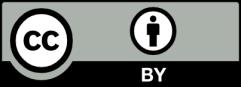