Open Access
ARTICLE
Generalized Jaccard Similarity Based Recurrent DNN for Virtualizing Social Network Communities
1 Department of Electronics and Communication Engineering, Hindusthan Institute of Technology, Coimbatore, 641050, Tamil Nadu, India
2Department of Electronics and Communication Engineering, Hindusthan College of Engineering and Technology, Coimbatore, 641032, Tamil Nadu, India
* Corresponding Author: R. Gnanakumari. Email:
Intelligent Automation & Soft Computing 2023, 36(3), 2719-2730. https://doi.org/10.32604/iasc.2023.034145
Received 07 July 2022; Accepted 27 August 2022; Issue published 15 March 2023
Abstract
In social data analytics, Virtual Community (VC) detection is a primary challenge in discovering user relationships and enhancing social recommendations. VC formation is used for personal interaction between communities. But the usual methods didn’t find the Suspicious Behaviour (SB) needed to make a VC. The Generalized Jaccard Suspicious Behavior Similarity-based Recurrent Deep Neural Network Classification and Ranking (GJSBS-RDNNCR) Model addresses these issues. The GJSBS-RDNNCR model comprises four layers for VC formation in Social Networks (SN). In the GJSBS-RDNNCR model, the SN is given as an input at the input layer. After that, the User’s Behaviors (UB) are extracted in the first Hidden Layer (HL), and the Generalized Jaccard Similarity coefficient calculates the similarity value at the second HL based on the SB. In the third HL, the similarity values are examined, and SB tendency is classified using the Activation Function (AF) in the Output Layer (OL). Finally, the ranking process is performed with classified users in SN and their SB. Results analysis is performed with metrics such as Classification Accuracy (CA), Time Complexity (TC), and False Positive Rate (FPR). The experimental setup considers 250 tweet users from the dataset to identify the SBs of users.Keywords
Cite This Article
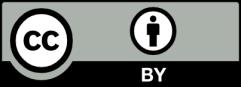
This work is licensed under a Creative Commons Attribution 4.0 International License , which permits unrestricted use, distribution, and reproduction in any medium, provided the original work is properly cited.