Open Access
ARTICLE
Deep Learning Driven Arabic Text to Speech Synthesizer for Visually Challenged People
1 King Salman Center for Disability Research, Riyadh, 13369, Saudi Arabia
2 Department of Information Technology, College of Computers and Information Technology, Taif University, P.O. Box 11099, Taif, 21944, Saudi Arabia
3 Department of Special Education, College of Education, King Saud University, Riyadh, 12372, Saudi Arabia
4 Department of Computer Science, College of Science & Arts at Muhayel, King Khaled University, Abha, 62217, Saudi Arabia
5 Department of Computer Science, College of Sciences and Humanities-Aflaj, Prince Sattam bin Abdulaziz University, Al-Aflaj, 16733, Saudi Arabia
6 Department of Computer and Self Development, Preparatory Year Deanship, Prince Sattam bin Abdulaziz University, AlKharj, 16242, Saudi Arabia
* Corresponding Author: Fahd N. Al-Wesabi. Email:
Intelligent Automation & Soft Computing 2023, 36(3), 2639-2652. https://doi.org/10.32604/iasc.2023.034069
Received 05 July 2022; Accepted 14 October 2022; Issue published 15 March 2023
Abstract
Text-To-Speech (TTS) is a speech processing tool that is highly helpful for visually-challenged people. The TTS tool is applied to transform the texts into human-like sounds. However, it is highly challenging to accomplish the TTS outcomes for the non-diacritized text of the Arabic language since it has multiple unique features and rules. Some special characters like gemination and diacritic signs that correspondingly indicate consonant doubling and short vowels greatly impact the precise pronunciation of the Arabic language. But, such signs are not frequently used in the texts written in the Arabic language since its speakers and readers can guess them from the context itself. In this background, the current research article introduces an Optimal Deep Learning-driven Arab Text-to-Speech Synthesizer (ODLD-ATSS) model to help the visually-challenged people in the Kingdom of Saudi Arabia. The prime aim of the presented ODLD-ATSS model is to convert the text into speech signals for visually-challenged people. To attain this, the presented ODLD-ATSS model initially designs a Gated Recurrent Unit (GRU)-based prediction model for diacritic and gemination signs. Besides, the Buckwalter code is utilized to capture, store and display the Arabic texts. To improve the TSS performance of the GRU method, the Aquila Optimization Algorithm (AOA) is used, which shows the novelty of the work. To illustrate the enhanced performance of the proposed ODLD-ATSS model, further experimental analyses were conducted. The proposed model achieved a maximum accuracy of 96.35%, and the experimental outcomes infer the improved performance of the proposed ODLD-ATSS model over other DL-based TSS models.Keywords
Cite This Article
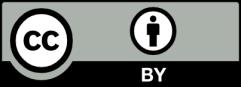