Open Access
ARTICLE
Multi-Path Attention Inverse Discrimination Network for Offline Signature Verification
1 School of Computer and Software, Nanjing University of Information Science & Technology, Nanjing, 210044, China
2 Wuxi Research Institute, Nanjing University of Information Science & Technology, Wuxi, 214100, China
3 Engineering Research Center of Digital Forensics, Ministry of Education, Jiangsu Engineering Center of Network Monitoring, Nanjing, 210044, China
4 Jiangsu Collaborative Innovation Center of Atmospheric Environment and Equipment Technology (CICAEET), Nanjing University of Information Science & Technology, Nanjing, 210044, China
5 School of Automation, Nanjing University of Information Science & Technology, Nanjing 210044, China
6 Department of Electrical and Computer Engineering, University of Windsor, Windsor, N9B 3P4, Canada
7 School of Teacher Education, Nanjing University of Information Science & Technology, Nanjing, 210044, China
* Corresponding Author: Xiaorui Zhang. Email:
Intelligent Automation & Soft Computing 2023, 36(3), 3057-3071. https://doi.org/10.32604/iasc.2023.033578
Received 21 June 2022; Accepted 22 September 2022; Issue published 15 March 2023
Abstract
Signature verification, which is a method to distinguish the authenticity of signature images, is a biometric verification technique that can effectively reduce the risk of forged signatures in financial, legal, and other business environments. However, compared with ordinary images, signature images have the following characteristics: First, the strokes are slim, i.e., there is less effective information. Second, the signature changes slightly with the time, place, and mood of the signer, i.e., it has high intraclass differences. These challenges lead to the low accuracy of the existing methods based on convolutional neural networks (CNN). This study proposes an end-to-end multi-path attention inverse discrimination network that focuses on the signature stroke parts to extract features by reversing the foreground and background of signature images, which effectively solves the problem of little effective information. To solve the problem of high intraclass variability of signature images, we add multi-path attention modules between discriminative streams and inverse streams to enhance the discriminative features of signature images. Moreover, a multi-path discrimination loss function is proposed, which does not require the feature representation of the samples with the same class label to be infinitely close, as long as the gap between inter-class distance and the intra-class distance is bigger than the set classification threshold, which radically resolves the problem of high intra-class difference of signature images. In addition, this loss can also spur the network to explore the detailed information on the stroke parts, such as the crossing, thickness, and connection of strokes. We respectively tested on CEDAR, BHSig-Bengali, BHSig-Hindi, and GPDS Synthetic datasets with accuracies of 100%, 96.24%, 93.86%, and 83.72%, which are more accurate than existing signature verification methods. This is more helpful to the task of signature authentication in justice and finance.Keywords
Cite This Article
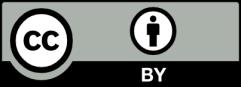
This work is licensed under a Creative Commons Attribution 4.0 International License , which permits unrestricted use, distribution, and reproduction in any medium, provided the original work is properly cited.