Open Access
ARTICLE
Enhanced Deep Learning for Detecting Suspicious Fall Event in Video Data
Department of CSE, University Institute of Technology, Rajiv Gandhi Proudyogiki Vishwavidyalaya, Bhopal, India
* Corresponding Author: Madhuri Agrawal. Email:
Intelligent Automation & Soft Computing 2023, 36(3), 2653-2667. https://doi.org/10.32604/iasc.2023.033493
Received 18 June 2022; Accepted 07 November 2022; Issue published 15 March 2023
Abstract
Suspicious fall events are particularly significant hazards for the safety of patients and elders. Recently, suspicious fall event detection has become a robust research case in real-time monitoring. This paper aims to detect suspicious fall events during video monitoring of multiple people in different moving backgrounds in an indoor environment; it is further proposed to use a deep learning method known as Long Short Term Memory (LSTM) by introducing visual attention-guided mechanism along with a bi-directional LSTM model. This method contributes essential information on the temporal and spatial locations of ‘suspicious fall’ events in learning the video frame in both forward and backward directions. The effective “You only look once V4” (YOLO V4)–a real-time people detection system illustrates the detection of people in videos, followed by a tracking module to get their trajectories. Convolutional Neural Network (CNN) features are extracted for each person tracked through bounding boxes. Subsequently, a visual attention-guided Bi-directional LSTM model is proposed for the final suspicious fall event detection. The proposed method is demonstrated using two different datasets to illustrate the efficiency. The proposed method is evaluated by comparing it with other state-of-the-art methods, showing that it achieves 96.9% accuracy, good performance, and robustness. Hence, it is acceptable to monitor and detect suspicious fall events.
Keywords
Cite This Article
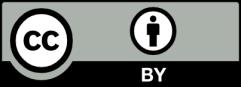
This work is licensed under a Creative Commons Attribution 4.0 International License , which permits unrestricted use, distribution, and reproduction in any medium, provided the original work is properly cited.