Open Access
ARTICLE
Adaptive Cyber Defense Technique Based on Multiagent Reinforcement Learning Strategies
1 Department of Cybersecurity, College of Computer Science and Engineering, University of Jeddah, Jeddah, Saudi Arabia
2 Department of Computer Science and Artificial Intelligence, College of Computer Science and Engineering, University of Jeddah, Jeddah, Saudi Arabia
* Corresponding Author: Adel Alshamrani. Email:
Intelligent Automation & Soft Computing 2023, 36(3), 2757-2771. https://doi.org/10.32604/iasc.2023.032835
Received 31 May 2022; Accepted 14 November 2022; Issue published 15 March 2023
Abstract
The static nature of cyber defense systems gives attackers a sufficient amount of time to explore and further exploit the vulnerabilities of information technology systems. In this paper, we investigate a problem where multiagent systems sensing and acting in an environment contribute to adaptive cyber defense. We present a learning strategy that enables multiple agents to learn optimal policies using multiagent reinforcement learning (MARL). Our proposed approach is inspired by the multiarmed bandits (MAB) learning technique for multiple agents to cooperate in decision making or to work independently. We study a MAB approach in which defenders visit a system multiple times in an alternating fashion to maximize their rewards and protect their system. We find that this game can be modeled from an individual player’s perspective as a restless MAB problem. We discover further results when the MAB takes the form of a pure birth process, such as a myopic optimal policy, as well as providing environments that offer the necessary incentives required for cooperation in multiplayer projects.Keywords
Cite This Article
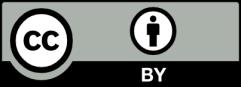
This work is licensed under a Creative Commons Attribution 4.0 International License , which permits unrestricted use, distribution, and reproduction in any medium, provided the original work is properly cited.