Open Access
ARTICLE
A Feature Learning-Based Model for Analyzing Students’ Performance in Supportive Learning
1 Department of Computer Science and Engineering, K. S. R College of Engineering, Thiruchengode, Tamil Nadu-637215, India
2 Centre for Artificial Intelligence, Chennai Institute of Technology, Chennai, Tamil Nadu-600069, India
3 Department of Computer Science and Engineering, S. A. Engineering College, Chennai, Tamil Nadu-600077, India
* Corresponding Author: P. Valarmathie. Email:
Intelligent Automation & Soft Computing 2023, 36(3), 2989-3005. https://doi.org/10.32604/iasc.2023.028659
Received 15 February 2022; Accepted 10 June 2022; Issue published 15 March 2023
Abstract
Supportive learning plays a substantial role in providing a quality education system. The evaluation of students’ performance impacts their deeper insight into the subject knowledge. Specifically, it is essential to maintain the baseline foundation for building a broader understanding of their careers. This research concentrates on establishing the students’ knowledge relationship even in reduced samples. Here, Synthetic Minority Oversampling TEchnique (SMOTE) technique is used for pre-processing the missing value in the provided input dataset to enhance the prediction accuracy. When the initial processing is not done substantially, it leads to misleading prediction accuracy. This research concentrates on modelling an efficient classifier model to predict students’ performance. Generally, the online available student dataset comprises a lesser amount of sample, and k-fold cross-validation is performed to balance the dataset. Then, the relationship among the students’ performance (features) is measured using the auto-encoder. The stacked Long Short Term Memory () is used to learn the previous feedback connection. The stacked model handles the provided data and the data sequence for understanding the long-term dependencies. The simulation is done in the MATLAB 2020a environment, and the proposed model shows a better trade-off than the existing approaches. Some evaluation metrics like prediction accuracy, sensitivity, specificity, AUROC, F1-score and recall are evaluated using the proposed model. The performance of the model is compared with existing approaches. The proposed model gives 89% accuracy, 83% precision, 86% recall, and 87% F-score. The proposed model outperforms the existing systems in terms of the earlier metrics.Keywords
Cite This Article
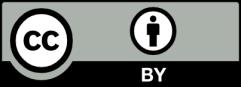
This work is licensed under a Creative Commons Attribution 4.0 International License , which permits unrestricted use, distribution, and reproduction in any medium, provided the original work is properly cited.