Open Access
ARTICLE
Deep Learning Framework for Landslide Severity Prediction and Susceptibility Mapping
Department of Computer Science and Engineering, SRM Institute of Science and Technology, Vadapalani Campus, 600026, Tamil Nadu, India
* Corresponding Author: G. Bhargavi. Email:
Intelligent Automation & Soft Computing 2023, 36(2), 1257-1272. https://doi.org/10.32604/iasc.2023.034335
Received 14 July 2022; Accepted 14 October 2022; Issue published 05 January 2023
Abstract
Landslides are a natural hazard that is unpredictable, but we can prevent them. The Landslide Susceptibility Index reduces the uncertainty of living with landslides significantly. Planning and managing landslide-prone areas is critical. Using the most optimistic deep neural network techniques, the proposed work classifies and analyses the severity of the landslide. The selected experimental study area is Kerala’s Idukki district. A total of 3363 points were considered for this experiment using historic landslide points, field surveys, and literature searches. The primary triggering factors slope degree, slope aspect, elevation (altitude), normalized difference vegetation index (NDVI), and distance from road, lithology, and rainfall are considered. A landslide susceptibility map was generated using the Arc geographic information system (GIS) tool for all the triggering factors using frequency ratio method, Shannon entropy method, Relative effect method, and fuzzy logic method. A new Deep Neural Network (DNN) framework has been developed for the multiclass classification and prediction of landslide hazard zones as low, moderate, high, and very high. Existing works are only uses statistical methods, but the proposed work has used DNN to predict landslide severity at four different level even for semi data with over accurately. The training data for deep learning model are generated using the Sentinel Satellite images and field survey. The label for training the data are generated from the Landslide Susceptibility Index which are generated from statistical method. Among all the statistical method generated data the Shannon Entropy data is the most accurate of the four statistical methods achieves 99.16%, accuracy. The frequency ratio method based data achieves 97.08% accuracy, the relative effect method based data achieves 92.72% accuracy, and the Fuzzy logic method based data achieves 86.60% accuracy.Keywords
Cite This Article
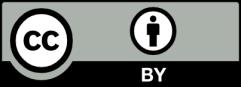
This work is licensed under a Creative Commons Attribution 4.0 International License , which permits unrestricted use, distribution, and reproduction in any medium, provided the original work is properly cited.