Open Access
ARTICLE
ProbD: Faulty Path Detection Based on Probability in Software-Defined Networking
1 School of Computer Science and Technology, Hainan University, Haikou, 570228, China
2 School of Software, Beijing Jiaotong University, Beijing, 10004, China
3 University of British Columbia, Vancouver, V5K1K5, Canada
* Corresponding Author: Deshun Li. Email:
Intelligent Automation & Soft Computing 2023, 36(2), 1783-1796. https://doi.org/10.32604/iasc.2023.034265
Received 12 July 2022; Accepted 27 August 2022; Issue published 05 January 2023
Abstract
With the increasing number of switches in Software-Defined Networking (SDN), there are more and more faults rising in the data plane. However, due to the existence of link redundancy and multi-path forwarding mechanisms, these problems cannot be detected in time. The current faulty path detection mechanisms have problems such as the large scale of detection and low efficiency, which is difficult to meet the requirements of efficient faulty path detection in large-scale SDN. Concerning this issue, we propose an efficient network path fault testing model ProbD based on probability detection. This model achieves a high probability of detecting arbitrary path fault in the form of small-scale random sampling. Under a certain path fault rate, ProbD obtains the curve of sample size and probability of detecting arbitrary path fault by randomly sampling network paths several times. After a small number of experiments, the ProbD model can correctly estimate the path fault rate of the network and calculate the total number of paths that need to be detected according to the different probability of detecting arbitrary path fault and the path fault rate of the network. The final experimental results show that, compared with the full path coverage test, the ProbD model based on probability detection can achieve efficient network testing with less overhead. Besides, the larger the network scale is, the more overhead will be saved.Keywords
Cite This Article
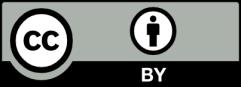
This work is licensed under a Creative Commons Attribution 4.0 International License , which permits unrestricted use, distribution, and reproduction in any medium, provided the original work is properly cited.