Open Access
ARTICLE
Bayes-Q-Learning Algorithm in Edge Computing for Waste Tracking
1 Department of Computer Science and Engineering, Dr. NGP Institute of Technology, Coimbatore, 641048, India
2 Department of Information Technology, Sri Krishna College of Technology, Coimbatore, 641042, India
3 Department of Computer Science, College of Computers and Information Technology, Taif University, P. O. Box 11099, Taif, 21944, Saudi Arabia
4 Department of Information Technology, College of Computers and Information Technology, Taif University, Taif P.O. Box 11099, Taif, 21944, Saudi Arabia
5 Department of Mathematics, Faculty of Science, Mansoura University, Mansoura, 35516, Egypt
6 Department of Computational Mathematics, Science, and Engineering (CMSE), Michigan State University, East Lansing, MI, 48824, USA
* Corresponding Author: Mohamed Abouhawwash. Email:
Intelligent Automation & Soft Computing 2023, 36(2), 2425-2440. https://doi.org/10.32604/iasc.2023.033879
Received 30 June 2022; Accepted 14 October 2022; Issue published 05 January 2023
Abstract
The major environmental hazard in this pandemic is the unhygienic disposal of medical waste. Medical wastage is not properly managed it will become a hazard to the environment and humans. Managing medical wastage is a major issue in the city, municipalities in the aspects of the environment, and logistics. An efficient supply chain with edge computing technology is used in managing medical waste. The supply chain operations include processing of waste collection, transportation, and disposal of waste. Many research works have been applied to improve the management of wastage. The main issues in the existing techniques are ineffective and expensive and centralized edge computing which leads to failure in providing security, trustworthiness, and transparency. To overcome these issues, in this paper we implement an efficient Naive Bayes classifier algorithm and Q-Learning algorithm in decentralized edge computing technology with a binary bat optimization algorithm (NBQ-BBOA). This proposed work is used to track, detect, and manage medical waste. To minimize the transferring cost of medical wastage from various nodes, the Q-Learning algorithm is used. The accuracy obtained for the Naïve Bayes algorithm is 88%, the Q-Learning algorithm is 82% and NBQ-BBOA is 98%. The error rate of Root Mean Square Error (RMSE) and Mean Error (MAE) for the proposed work NBQ-BBOA are 0.012 and 0.045.Keywords
Cite This Article
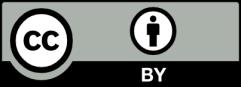
This work is licensed under a Creative Commons Attribution 4.0 International License , which permits unrestricted use, distribution, and reproduction in any medium, provided the original work is properly cited.