Open Access
ARTICLE
Early Detection Glaucoma and Stargardt’s Disease Using Deep Learning Techniques
Department of Electronics and Communication Engineering, Sri Shakthi Institute of Engineering and Technology, Coimabatore, 641062, Tamilnadu, India
* Corresponding Author: Somasundaram Devaraj. Email:
Intelligent Automation & Soft Computing 2023, 36(2), 1283-1299. https://doi.org/10.32604/iasc.2023.033200
Received 10 June 2022; Accepted 02 August 2022; Issue published 05 January 2023
Abstract
Retinal fundus images are used to discover many diseases. Several Machine learning algorithms are designed to identify the Glaucoma disease. But the accuracy and time consumption performance were not improved. To address this problem Max Pool Convolution Neural Kuan Filtered Tobit Regressive Segmentation based Radial Basis Image Classifier (MPCNKFTRS-RBIC) Model is used for detecting the Glaucoma and Stargardt’s disease by early period using higher accuracy and minimal time. In MPCNKFTRS-RBIC Model, the retinal fundus image is considered as an input which is preprocessed in hidden layer 1 using weighted adaptive Kuan filter. Then, preprocessed retinal fundus is given for hidden layer 2 for extracting the features like color, intensity, texture with higher accuracy. After extracting these features, the Tobit Regressive Segmentation process is performed by hidden layer 3 for partitioning preprocessed image within more segments by analyzing the pixel with the extracted features of the fundus image. Then, the segmented image was given to output layer. The radial basis function analyzes the testing image region of a particular class as well as training image region with higher accuracy and minimum time consumption. Simulation is performed with retinal fundus image dataset with various performance metrics namely peak signal-to-noise ratio, accuracy and time, error rate concerning several retina fundus image and image size.Keywords
Cite This Article
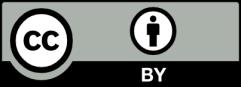
This work is licensed under a Creative Commons Attribution 4.0 International License , which permits unrestricted use, distribution, and reproduction in any medium, provided the original work is properly cited.