Open Access
ARTICLE
Novel Multimodal Biometric Feature Extraction for Precise Human Identification
1 Department of Computer Science and Engineering, SRM Institute of Science and Technology, Kattankulathur, 603203, Tamil Nadu, India
2 Department of Computational Intelligence, SRM Institute of Science and Technology, Kattankulathur, 603203, Tamil Nadu, India
* Corresponding Author: M. S. Abirami. Email:
Intelligent Automation & Soft Computing 2023, 36(2), 1349-1363. https://doi.org/10.32604/iasc.2023.032604
Received 23 May 2022; Accepted 08 July 2022; Issue published 05 January 2023
Abstract
In recent years, biometric sensors are applicable for identifying important individual information and accessing the control using various identifiers by including the characteristics like a fingerprint, palm print, iris recognition, and so on. However, the precise identification of human features is still physically challenging in humans during their lifetime resulting in a variance in their appearance or features. In response to these challenges, a novel Multimodal Biometric Feature Extraction (MBFE) model is proposed to extract the features from the noisy sensor data using a modified Ranking-based Deep Convolution Neural Network (RDCNN). The proposed MBFE model enables the feature extraction from different biometric images that includes iris, palm print, and lip, where the images are preprocessed initially for further processing. The extracted features are validated after optimal extraction by the RDCNN by splitting the datasets to train the feature extraction model and then testing the model with different sets of input images. The simulation is performed in matlab to test the efficacy of the modal over multi-modal datasets and the simulation result shows that the proposed method achieves increased accuracy, precision, recall, and F1 score than the existing deep learning feature extraction methods. The performance improvement of the MBFE Algorithm technique in terms of accuracy, precision, recall, and F1 score is attained by 0.126%, 0.152%, 0.184%, and 0.38% with existing Back Propagation Neural Network (BPNN), Human Identification Using Wavelet Transform (HIUWT), Segmentation Methodology for Non-cooperative Recognition (SMNR), Daugman Iris Localization Algorithm (DILA) feature extraction techniques respectively.Keywords
Cite This Article
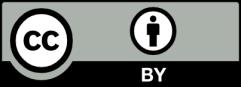