Open Access
ARTICLE
Multi Class Brain Cancer Prediction System Empowered with BRISK Descriptor
Department of Electronics and Communication Engineering, VISTAS, Chennai, Tamil Nadu, India
* Corresponding Author: Madona B. Sahaai. Email:
Intelligent Automation & Soft Computing 2023, 36(2), 1507-1521. https://doi.org/10.32604/iasc.2023.032256
Received 11 May 2022; Accepted 06 July 2022; Issue published 05 January 2023
Abstract
Magnetic Resonance Imaging (MRI) is one of the important resources for identifying abnormalities in the human brain. This work proposes an effective Multi-Class Classification (MCC) system using Binary Robust Invariant Scalable Keypoints (BRISK) as texture descriptors for effective classification. At first, the potential Region Of Interests (ROIs) are detected using features from the accelerated segment test algorithm. Then, non-maxima suppression is employed in scale space based on the information in the ROIs. The discriminating power of BRISK is examined using three machine learning classifiers such as k-Nearest Neighbour (kNN), Support Vector Machine (SVM) and Random Forest (RF). An MCC system is developed which classifies the MRI images into normal, glioma, meningioma and pituitary. A total of 3264 MRI brain images are employed in this study to evaluate the proposed MCC system. Results show that the average accuracy of the proposed MCC-RF based system is 99.62% with a sensitivity of 99.16% and specificity of 99.75%. The average accuracy of the MCC-kNN system is 93.65% and 97.59% by the MCC-SVM based system.Keywords
Cite This Article
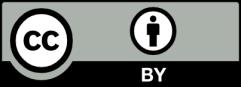
This work is licensed under a Creative Commons Attribution 4.0 International License , which permits unrestricted use, distribution, and reproduction in any medium, provided the original work is properly cited.