Open Access
ARTICLE
EliteVec: Feature Fusion for Depression Diagnosis Using Optimized Long Short-Term Memory Network
Mepco Schlenk Engineering College (Autonomous), Sivakasi, 626005, Tamilnadu, India
* Corresponding Author: S. Kavi Priya. Email:
Intelligent Automation & Soft Computing 2023, 36(2), 1745-1766. https://doi.org/10.32604/iasc.2023.032160
Received 09 May 2022; Accepted 17 August 2022; Issue published 05 January 2023
Abstract
Globally, depression is perceived as the most recurrent and risky disorder among young people and adults under the age of 60. Depression has a strong influence on the usage of words which can be observed in the form of written texts or stories posted on social media. With the help of Natural Language Processing(NLP) and Machine Learning (ML) techniques, the depressive signs expressed by people can be identified at the earliest stage from their Social Media posts. The proposed work aims to introduce an efficacious depression detection model unifying an exemplary feature extraction scheme and a hybrid Long Short-Term Memory network (LSTM) model. The feature extraction process combines a novel feature selection method called Elite Term Score (ETS) and Word2Vec to extract the syntactic and semantic information respectively. First, the ETS method leverages the document level, class level, and corpus level probabilities for computing the weightage/score of the terms. Then, the ideal and pertinent set of features with a high ETS score is selected, and the Word2vec model is trained to generate the intense feature vector representation for the set of selected terms. Finally, the resultant word vector obtained is called EliteVec, which is fed to the hybrid LSTM model based on Honey Badger optimizer with population reduction technique (PHB) which predicts whether the input textual content is depressive or not. The PHB algorithm is integrated to explore and exploit the optimal hyperparameters for strengthening the performance of the LSTM network. The comprehensive experiments are carried out with two different Twitter depression corpus based on accuracy and Root Mean Square Error (RMSE) metrics. The results demonstrated that the proposed EliteVec+LSTM+PHB model outperforms the state-of-art models with 98.1% accuracy and 0.0559 RMSE.Keywords
Cite This Article
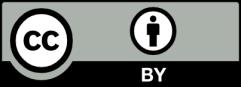
This work is licensed under a Creative Commons Attribution 4.0 International License , which permits unrestricted use, distribution, and reproduction in any medium, provided the original work is properly cited.