Open Access
ARTICLE
Auxiliary Classifier of Generative Adversarial Network for Lung Cancer Diagnosis
1 Department of Electrical and Electronics Engineering, Panimalar Institute of Technology, Chennai, Tamil Nadu, India
2 Department of Electronics and Communication Engineering, Sri Indu College of Engineering and Technology, Hyderabad, India
3 Department of Electronics and Communication Engineering, Sri Chandrasekharendra Saraswathi Viswa Mahavidyalaya University, (Deemed University), Kanchipuram, Tamil Nadu, India
4 Electronics and Communication Engineering, Saveetha School of Engineering, Saveetha Institute of Medical and Technical Sciences, Chennai, Tamil Nadu, India
* Corresponding Author: P. S. Ramapraba. Email:
Intelligent Automation & Soft Computing 2023, 36(2), 2177-2189. https://doi.org/10.32604/iasc.2023.032040
Received 04 May 2022; Accepted 15 June 2022; Issue published 05 January 2023
Abstract
The classification of lung nodules is a challenging problem as the visual analysis of the nodules and non-nodules revealed homogenous textural patterns. In this work, an Auxiliary Classifier (AC)-Generative Adversarial Network (GAN) based Lung Cancer Classification (LCC) system is developed. The proposed AC-GAN-LCC system consists of three modules; preprocessing, Lungs Region Detection (LRD), and AC-GAN classification. A Wiener filter is employed in the preprocessing module to remove the Gaussian noise. In the LRD module, only the lung regions (left and right lungs) are detected using iterative thresholding and morphological operations. In order to extract the lung region only, flood filling and background subtraction. The detected lung regions are fed to the AC-GAN classifier to detect the nodules. It classifies the nodules into one of the two classes, i.e., binary classification (such as nodules or non-nodules). The AC-GAN is the extended version of the conditional GAN that predicts the label of a given image. Three different optimization techniques, adaptive gradient optimization, root mean square propagation optimization, and Adam optimization are employed for optimizing the AC-GAN architecture. The proposed AC-GAN-LCC system is evaluated on the Lung Image Database Consortium (LIDC) database Computed Tomography (CT) scan images. The proposed AC-GAN-LCC system classifies ∼15000 CT slices (7310 non-nodules and 7685 nodules). It provides an overall accuracy of 98.8% on the LIDC database using Adam optimization by a 10-fold cross-validation approach.Keywords
Cite This Article
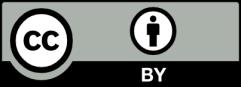
This work is licensed under a Creative Commons Attribution 4.0 International License , which permits unrestricted use, distribution, and reproduction in any medium, provided the original work is properly cited.