Open Access
ARTICLE
A Hybrid Deep Learning Model for Real Time Hand Gestures Recognition
1 Department of Information Technology, Easwari Engineering College, Chennai, 600089, India
2 Department of Electronics and Communication Engineering, Easwari Engineering College, Chennai, 600089, India
* Corresponding Author: S. Gnanapriya. Email:
Intelligent Automation & Soft Computing 2023, 36(1), 1105-1119. https://doi.org/10.32604/iasc.2023.032832
Received 31 May 2022; Accepted 05 July 2022; Issue published 29 September 2022
Abstract
The performance of Hand Gesture Recognition (HGR) depends on the hand shape. Segmentation helps in the recognition of hand gestures for more accuracy and improves the overall performance compared to other existing deep neural networks. The crucial segmentation task is extremely complicated because of the background complexity, variation in illumination etc. The proposed modified UNET and ensemble model of Convolutional Neural Networks (CNN) undergoes a two stage process and results in proper hand gesture recognition. The first stage is segmenting the regions of the hand and the second stage is gesture identification. The modified UNET segmentation model is trained using resized images to generate a cost effective semantic segmentation model. The Central Processing Unit (CPU) utilization and training time taken by these models with respect to three public benchmark datasets are also analyzed. Recognition is carried out with the ensemble learning model consisting of EfficientNet B0, EfficientNet B4 and ResNet V2 152. Experimentation on NUS hand posture dataset-II, OUHANDS and HGRI benchmark datasets show that our architecture achieves a maximum recognition rate of 99.07% through semantic segmentation and the Ensemble learning model.Keywords
Cite This Article
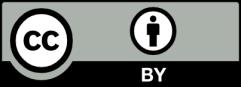