Open Access
ARTICLE
Stacking Ensemble Learning-Based Convolutional Gated Recurrent Neural Network for Diabetes Miletus
1 Sathyabama Institute of Science and Technology, 603202, Tamilnadu, India
2 SRM Institute of Science and Technology, 603202, Tamilnadu, India
* Corresponding Author: G. Geetha. Email:
Intelligent Automation & Soft Computing 2023, 36(1), 703-718. https://doi.org/10.32604/iasc.2023.032530
Received 21 May 2022; Accepted 06 July 2022; Issue published 29 September 2022
Abstract
Diabetes mellitus is a metabolic disease in which blood glucose levels rise as a result of pancreatic insulin production failure. It causes hyperglycemia and chronic multiorgan dysfunction, including blindness, renal failure, and cardiovascular disease, if left untreated. One of the essential checks that are needed to be performed frequently in Type 1 Diabetes Mellitus is a blood test, this procedure involves extracting blood quite frequently, which leads to subject discomfort increasing the possibility of infection when the procedure is often recurring. Existing methods used for diabetes classification have less classification accuracy and suffer from vanishing gradient problems, to overcome these issues, we proposed stacking ensemble learning-based convolutional gated recurrent neural network (CGRNN) Metamodel algorithm. Our proposed method initially performs outlier detection to remove outlier data, using the Gaussian distribution method, and the Box-cox method is used to correctly order the dataset. After the outliers’ detection, the missing values are replaced by the data’s mean rather than their elimination. In the stacking ensemble base model, multiple machine learning algorithms like Naïve Bayes, Bagging with random forest, and Adaboost Decision tree have been employed. CGRNN Meta model uses two hidden layers Long-Short-Time Memory (LSTM) and Gated Recurrent Unit (GRU) to calculate the weight matrix for diabetes prediction. Finally, the calculated weight matrix is passed to the softmax function in the output layer to produce the diabetes prediction results. By using LSTM-based CG-RNN, the mean square error (MSE) value is 0.016 and the obtained accuracy is 91.33%.Keywords
Cite This Article
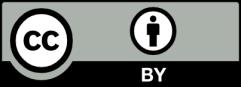
This work is licensed under a Creative Commons Attribution 4.0 International License , which permits unrestricted use, distribution, and reproduction in any medium, provided the original work is properly cited.