Open Access
ARTICLE
Machine Learning-Based Models for Magnetic Resonance Imaging (MRI)-Based Brain Tumor Classification
1 Department of Radiological Sciences, College of Applied Medical Sciences, Najran University, Najran, Saudi Arabia
2 Department of Computer Science, City University of Science and Information Technology, Peshawar, Pakistan
3 Department of Electrical Engineering, University of Engineering and Technology, Mardan, 23200, Pakistan
4 Department of Computer Science Engineering, University of Engineering and Technology, Mardan, 23200, Pakistan
5 Electrical Engineering Department, College of Engineering, Najran University Saudi Arabia, Najran, 61441, Saudi Arabia
* Corresponding Author: Fazal Muhammad. Email:
Intelligent Automation & Soft Computing 2023, 36(1), 299-312. https://doi.org/10.32604/iasc.2023.032426
Received 17 May 2022; Accepted 22 June 2022; Issue published 29 September 2022
Abstract
In the medical profession, recent technological advancements play an essential role in the early detection and categorization of many diseases that cause mortality. The technique rising on daily basis for detecting illness in magnetic resonance through pictures is the inspection of humans. Automatic (computerized) illness detection in medical imaging has found you the emergent region in several medical diagnostic applications. Various diseases that cause death need to be identified through such techniques and technologies to overcome the mortality ratio. The brain tumor is one of the most common causes of death. Researchers have already proposed various models for the classification and detection of tumors, each with its strengths and weaknesses, but there is still a need to improve the classification process with improved efficiency. However, in this study, we give an in-depth analysis of six distinct machine learning (ML) algorithms, including Random Forest (RF), Naïve Bayes (NB), Neural Networks (NN), CN2 Rule Induction (CN2), Support Vector Machine (SVM), and Decision Tree (Tree), to address this gap in improving accuracy. On the Kaggle dataset, these strategies are tested using classification accuracy, the area under the Receiver Operating Characteristic (ROC) curve, precision, recall, and F1 Score (F1). The training and testing process is strengthened by using a 10-fold cross-validation technique. The results show that SVM outperforms other algorithms, with 95.3% accuracy.Keywords
Cite This Article
A. A. Asiri, B. Khan, F. Muhammad, S. Ur Rahman, H. A. Alshamrani et al., "Machine learning-based models for magnetic resonance imaging (mri)-based brain tumor classification," Intelligent Automation & Soft Computing, vol. 36, no.1, pp. 299–312, 2023. https://doi.org/10.32604/iasc.2023.032426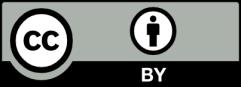